Imagine this: you browse vacation deals, and within minutes, ads for flights and hotels follow you across every app and website. Convenient? Maybe. Creepy? Absolutely.
This is the paradox of marketing personalisation. Research from Epsilon revealed that 80% of consumers are more likely to purchase when brands offer tailored experiences. Yet, Gartner warned that over half of consumers would unsubscribe from communications, and 38% would stop doing business with a brand if personalisation crossed into invasive territory.
The stakes are high. Missteps can drive customers away, while thoughtful personalisation fosters trust and loyalty. So, where do marketers draw the line between relevant and invasive?
At the heart of data-driven campaigns lies a fundamental question: how personal is too personal? Modern consumers crave relevance but fiercely guard their privacy. Personalisation helps brands deliver the right message at the right time. However, when data collection feels excessive or targeting becomes intrusive, it can erode the very trust it aims to build.
The Promise and Perils of Personalisation
Personalisation is an expectation. Consumers want brands to understand their preferences, anticipate their needs, and deliver uniquely tailored experiences. A well-timed recommendation or a curated shopping experience can create a connection that drives loyalty. According to McKinsey, 71% of consumers now expect personalised interactions from brands, and 76% feel frustrated when this expectation isn’t met.
However, there’s a thin line between thoughtful targeting and overstepping. When personalisation is done poorly—such as overly aggressive retargeting or eerily precise ads—it can leave consumers feeling watched rather than understood. Sometimes, these efforts backfire entirely, damaging the brand’s reputation and alienating its audience.
Take the infamous case of Target’s predictive analytics. By analyzing purchase data, the retailer could identify likely pregnant shoppers. While the campaign was a testament to the power of data, it drew widespread criticism for being invasive after a father received maternity ads meant for his teenage daughter. This backlash underscored the risks of crossing the personalisation threshold.
Consumers appreciate relevance, but only when it comes with respect for their privacy. Brands that fail to navigate this balance risk losing trust, an increasingly difficult commodity to regain. The challenge lies in creating personalised experiences that add value without compromising the consumer’s sense of control.
As the debate around personalisation intensifies, one thing is clear: understanding where to draw the line is essential for long-term success.
Case Studies: Striking the Balance in Personalisation
Spotify Wrapped, A Celebration of Individuality
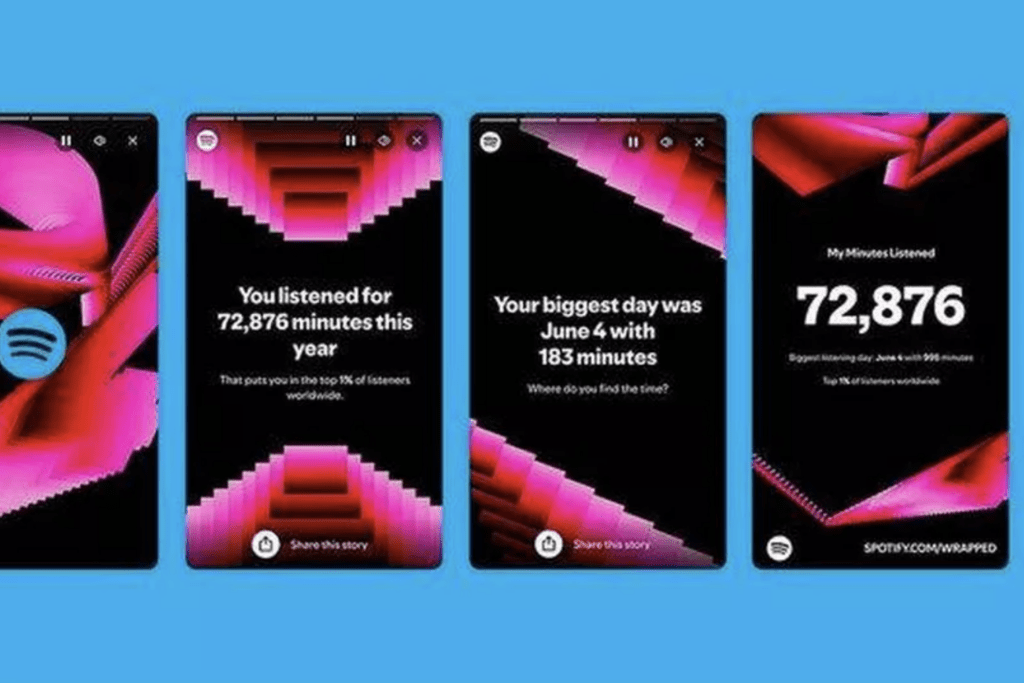
Image Credit: Yorkshire Live
Background
Spotify’s annual Wrapped campaign has become a cultural phenomenon. It leverages user data to create highly personalised year-in-review summaries. By analyzing individual listening habits, Spotify delivers curated insights that resonate with users on a personal level.
Approach
Wrapped provides users with data points like their most-streamed songs, favourite genres, and total listening hours. The key lies in Spotify’s transparency—users are aware of the collected data and how it’s used to enhance their experience. Wrapped feels less like a marketing tool and more like a celebration of personal tastes, encouraging users to share their unique results on social media and amplifying the campaign’s reach organically.
Outcomes
Spotify Wrapped consistently generates widespread engagement, with millions of users sharing their results online. This reinforces brand loyalty and attracts new subscribers through the campaign’s viral appeal. By striking the right balance between personalisation and privacy, Spotify exemplifies how brands can use data to enhance customer relationships.
Apple’s Privacy-First Personalisation
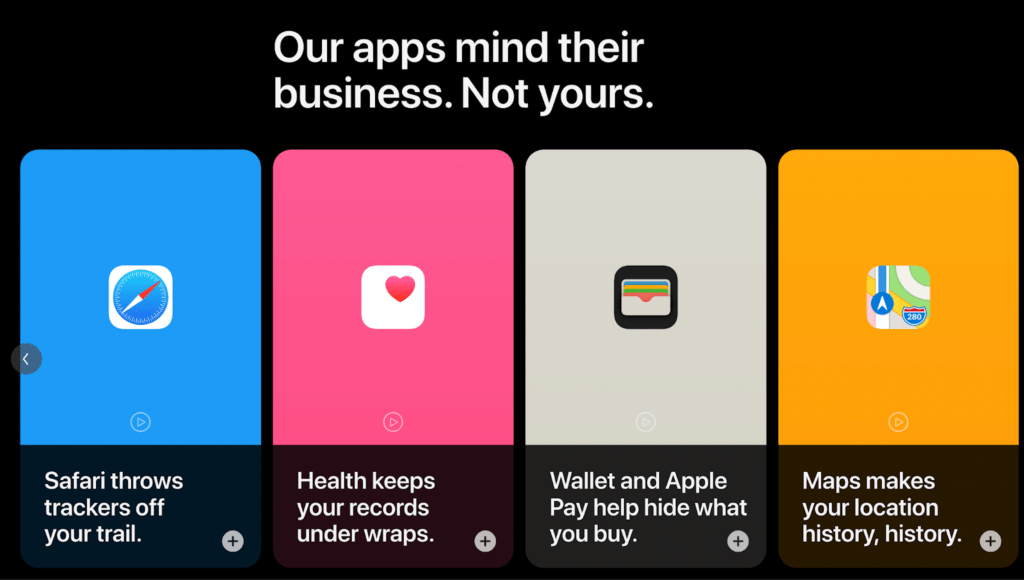
Image Credit: Apple
Background
As consumer concerns over data privacy have grown, Apple has positioned itself as a leader in protecting user information. Through its App Tracking Transparency (ATT) feature, Apple gives users greater control over how their data is shared, redefining the boundaries of personalisation.
Approach
Instead of relying on third-party data, Apple employs on-device intelligence for personalisation. Features like Siri suggestions, curated news, and photo memories use data stored locally on the user’s device. This ensures personalisation without compromising privacy. Apple has also used its marketing to reinforce its stance, making privacy a key selling point.
Outcomes
Apple’s approach has bolstered consumer trust and differentiated the brand in a crowded market. By emphasising user consent and privacy, Apple complies with evolving regulations and aligns with consumer expectations for ethical data use. The success of this strategy is evident in its customer retention and the loyalty of privacy-conscious users.
Building Trust Through Ethical Personalisation
The foundation of effective personalisation lies in balancing relevance with respect for privacy. To foster trust and loyalty, brands must adopt ethical practices prioritising consumer consent, transparency, and meaningful engagement. Here are key strategies, supported by real-world examples, to achieve this balance:
- Prioritise Transparency and Consent
Consumers value honesty. Clearly communicate how data is collected, stored, and used. Providing opt-in mechanisms and user-friendly privacy policies empowers consumers to make informed choices.
For example, Apple’s App Tracking Transparency feature explicitly asks users for consent to track their activity across apps. This approach has set a new standard for privacy-first personalisation, building trust while maintaining relevance.
- Use Data to Address Consumer Needs
Personalisation works best when it solves real pain points or enhances the user experience. Focus on delivering relevant, value-driven interactions rather than excessive targeting.
For instance, Netflix uses viewer history to recommend content tailored to individual tastes. This non-intrusive personalisation creates a seamless experience that keeps users engaged without overstepping boundaries.
- Embrace Privacy-Enhancing Technologies
Emerging technologies like federated learning and edge computing enable brands to deliver personalised experiences while safeguarding user data. These tools process data locally on devices, reducing the risks of breaches and misuse.
Google’s federated learning model, used for improving predictive text features, demonstrates how personalisation can be achieved without compromising user privacy or centralising sensitive information.
- Tailor Campaigns to Regional and Cultural Preferences
Personalisation is not one-size-fits-all. Consider cultural norms and regional differences when designing campaigns to ensure they resonate with diverse audiences.
For example, in Japan, subtlety and discretion are highly valued in marketing, while in Southeast Asia, interactive campaigns that offer clear value, such as discounts or rewards, are more effective. Brands like Grab, a ride-hailing and delivery service in Southeast Asia, personalise their offers based on local events and consumer habits, enhancing engagement across diverse markets.
- Involve Consumers in the Personalisation Process
Co-creating personalised experiences by inviting consumers to set their preferences fosters a sense of control and reduces privacy concerns.
For example, streaming platforms like YouTube let users set preferences for recommended content through thumbs-up or thumbs-down features. This approach ensures users feel more in control of their experience while improving the relevance of future recommendations.
- Monitor and Adapt to Feedback
Consumer expectations evolve, and so should personalisation strategies. Regularly gathering feedback through surveys, reviews, and sentiment analysis can help brands refine their approach.
For example, brands that adapt their email marketing frequency or content style based on user feedback often see higher engagement rates and fewer unsubscribes.
Lessons from Global Markets
United States: The Demand for Transparency
In the United States, consumer awareness of data privacy is at an all-time high, driven by legislation like the California Consumer Privacy Act (CCPA) and high-profile data breaches. Brands operating in this market are under pressure to provide clear, user-friendly privacy policies and secure consent mechanisms. Companies like Apple have capitalised on this trend, making privacy a cornerstone of their brand narrative and setting a high bar for competitors.
Europe: Privacy Regulations as a Benchmark
Europe’s General Data Protection Regulation (GDPR) has become a global standard for privacy compliance. Brands in the EU face strict rules about data collection, storage, and usage. Successful examples include local e-commerce platforms that explicitly inform users about tracking cookies and provide clear opt-in choices. These efforts have ensured compliance and fostered greater trust among European consumers.
Asia: Personalisation Across Diverse Cultures
Asia’s markets present unique challenges due to their cultural diversity and varying attitudes toward privacy. For instance, personalisation efforts in Japan often emphasise subtlety and respect for privacy, aligning with cultural norms. In contrast, Southeast Asian consumers, particularly in countries like Indonesia and the Philippines, tend to engage more enthusiastically with data-driven campaigns, provided they see clear value in return.
Global brands like Netflix have tailored their strategies to these nuances, offering region-specific content recommendations that respect local tastes while maintaining transparency. Such localised personalisation efforts can enhance engagement while avoiding a one-size-fits-all approach.
Takeaways for Brands
The differing expectations across regions highlight the importance of understanding local market dynamics. Brands looking to implement ethical personalisation globally must align their strategies with each market’s cultural and regulatory landscape. By respecting regional preferences and adhering to privacy standards, they can create personalised experiences that resonate without overstepping boundaries.
The Future of Personalisation
As technology evolves, so will the possibilities and challenges of personalisation. Emerging trends and innovations are already reshaping how brands approach tailored marketing, raising new questions about ethics, privacy, and consumer trust.
AI-Powered Personalisation
Artificial intelligence is driving the next wave of hyper-personalisation, enabling brands to predict consumer behaviour with unprecedented accuracy. Machine learning models analyze vast amounts of data to offer real-time recommendations, from product suggestions to personalised content. However, as these systems become more advanced, the risk of appearing overly invasive increases, underscoring the need for ethical guardrails in AI deployment.
Zero-Party Data Strategies
With consumers becoming more cautious about sharing their information, brands are turning to zero-party data, information that customers willingly provide. This approach emphasises transparency and gives consumers control over their data, making personalisation a collaboration rather than an imposition. Interactive tools like quizzes, preference centres, and surveys allow brands to gather valuable insights while building trust.
Contextual Personalisation Without Tracking
Advancements in contextual targeting are paving the way for personalisation that doesn’t rely on tracking individual users. By analyzing environmental factors such as location, weather, or time of day, brands can deliver relevant messages without compromising privacy. For example, a food delivery app might promote comfort foods on rainy days based on real-time weather data rather than user profiles.
Stronger Privacy Regulations
The rise of privacy-focused legislation worldwide pushes brands to rethink how they collect and use data. Markets with stringent privacy laws, like the European Union and California, are setting precedents that other regions are beginning to follow. Brands that proactively adapt to these changes and invest in compliance and privacy-first technologies will gain a competitive edge.
A Shift Toward Ethical Personalisation
Consumer demand for responsible data use is driving the push for ethical personalisation. Organisations like the World Economic Forum call for global standards that balance innovation with privacy. Brands that adopt these principles early will not only stay ahead of regulatory changes but also solidify their position as consumer-first businesses.
The future of personalisation lies in achieving the right balance between technology and ethics. As data collection becomes more sophisticated and consumer expectations rise, brands that walk the fine line between relevance and privacy will emerge as leaders.
Effective personalisation isn’t about amassing more data but using it responsibly. Campaigns rooted in transparency, respect for privacy, and genuine value will foster trust. Brands focusing on connection over surveillance and relevance over excess will thrive.
The question for marketers isn’t just how to personalise but how to do it in a way that earns trust and strengthens relationships. As consumers demand relevance and respect, the true test for brands will be whether they can deliver personalisation with purpose.
Get regular insights
Keep up to date with the latest insights from our research as well as all our company news in our free monthly newsletter.
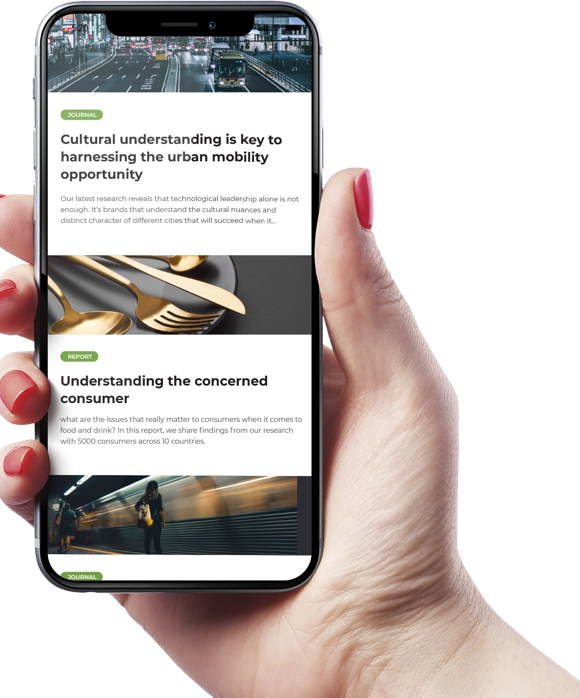