Imagine a consumer, let’s call her Sarah, standing in the grocery store aisle, trying to choose a breakfast cereal. She has many options: some are low in sugar, others boast high fibre content, and others are flavoured or branded with popular characters. Each of these attributes represents a potential factor in Sarah’s decision-making process. The research methodology called Discrete Choice Modelling (DCM) seeks to understand and quantify the influence of these factors on her choice.
Sarah’s decision is not random but rather a complex interplay of preferences and trade-offs. She might prioritise health over flavour or be willing to pay a little extra for a brand she trusts. Discrete Choice Modelling helps break down this decision-making process into quantifiable elements, allowing brands to analyse which factors sway the consumer’s choice most.
Understanding Consumer Decisions
Discrete Choice Modelling is a critical tool in market research and consumer behaviour analysis. DCM is a statistical technique used to predict consumer choices and preferences. It operates on the principle that consumers make decisions by evaluating various alternatives and selecting the one that best suits their needs and preferences. This model breaks down the decision-making process into discrete choices, making it easier to analyse and understand.
Understanding consumer preferences is essential in a fiercely competitive and rapidly changing market. Brands that grasp what drives their customers’ choices can create more effective marketing strategies, develop products that resonate with their target audience, and ultimately achieve a stronger market position. By leveraging DCM, companies can gain insights into consumer decision-making’s often complex and multifaceted nature.
This approach to understanding consumer behaviour is particularly relevant when customer expectations continuously evolve. With the advent of digital technology and social media, consumers are more informed and have higher expectations. They are bombarded with choices, and many factors, including brand image, product features, price, and peer reviews, can influence their preferences. Discrete Choice Modelling helps brands decode these preferences by providing a structured way to analyse consumers’ trade-offs.
So, how can brands effectively implement this technique to stay ahead in understanding and catering to their customer base?
The Science Behind Discrete Choice Modelling (DCM)
Discrete Choice Modelling is about understanding and predicting choices. The method operates under the assumption that when individuals are faced with multiple options, their selection is influenced by the characteristics of these options. DCM models decision-making by identifying these attributes and assessing their impact on the individual’s choice.
The Mathematical Foundation: Probability Meets Attributes
The core of DCM lies in its mathematical foundation, which hinges on the relationship between probability and the attributes of choices. The method uses statistical models to estimate the likelihood of a consumer choosing one option over others based on the characteristics of each choice. For example, in our earlier example of Sarah choosing breakfast cereal, DCM would assign probabilities to her choosing each cereal based on attributes like sugar content, fibre content, flavour, and brand.
This probability is not a straightforward calculation; it is a complex estimation considering how each attribute influences the decision. The model might reveal, for instance, that a 10% decrease in sugar content increases the probability of choosing a particular cereal by 5%. These kinds of insights are obtained by analysing data from actual consumer choices, often collected through surveys or observed purchasing behaviour.
The statistical techniques used in DCM can vary. Still, they often involve regression models where the dependent variable is the choice made, and the independent variables are the attributes of the products. The goal is to estimate the parameters that best describe how these attributes are weighed in decision-making.
One of the most commonly used models in DCM is the Multinomial Logit Model, which provides a way to estimate the probability of each choice as a function of its attributes and some random component that captures unobserved factors. This model is particularly favoured for its simplicity and interpretability.
Through these mathematical models, DCM provides a framework to quantify how changes in product attributes can influence consumer choices. This quantification is powerful, allowing brands to forecast how variations in product features or marketing strategies might shift consumer preferences and market dynamics.
Key Components of Discrete Choice Modelling (DCM)
Alternatives: The Range of Options
One of the fundamental elements of DCM is the concept of ‘alternatives.’ These are the different options available to the customer. In any given choice scenario, consumers are presented with a set of alternatives from which they select one. Let’s use Sarah’s example where she is looking for cereal. Sarah is deciding on her breakfast choice for the morning.
Alternatives: She has several breakfast options, each varying in nutritional content, taste, preparation time, and other attributes. These options might include:
- Cereal A: A high-fiber, low-sugar cereal known for its health benefits but with a relatively plain taste.
- Cereal B: A sweet, flavoured cereal with added vitamins, appealing to those looking for a tastier option but with higher sugar content.
- Cereal C: An organic cereal, slightly more expensive, offering natural ingredients and no artificial flavours.
- Cereal D: A convenient, pre-packaged cereal bar, ideal for on-the-go consumption but potentially less nutritious than other options.
In this scenario, each cereal (A, B, C, D) represents a different alternative for Sarah’s breakfast. She will consider various factors such as healthiness, taste preferences, budget, and convenience when making her choice. Discrete Choice Modelling would analyse factors like these to predict which breakfast option Sarah will most likely choose based on each alternative’s specific attributes.
This shows how consumers evaluate different options based on characteristics and how these evaluations influence their ultimate decision.
Attributes: Defining Characteristics
The second key component of DCM is ‘attributes,’ which are each alternative’s specific characteristics or benefits. Attributes are the factors that consumers evaluate when making a decision. They can be tangible, like price or size, or intangible, like brand reputation or perceived quality. In the cereal example, attributes might include sugar content, fibre content, flavour, price, packaging, and brand. It is these attributes that consumers weigh against each other when making their choice.
Utility: The Customer’s Perceived Value
The third critical component is ‘utility.’ This refers to the value a customer places on each attribute of the alternatives. In DCM, utility measures a consumer’s satisfaction or benefit from choosing a particular alternative. It’s a complex concept because it encompasses not just the objective attributes of a product but also the subjective preferences and perceptions of the consumer.
Utility is often modeled as a function of the attributes of the alternatives. For example, one consumer might place high utility on low sugar content due to health considerations, while another might value flavour more. The utility function in DCM attempts to capture these preferences, allowing for the prediction of which alternative a consumer is most likely to choose.
Applications and Benefits of Discrete Choice Modelling
Optimising Product Features with DCM
Brands use Discrete Choice Modelling as a strategic tool to fine-tune their product features, aligning them more closely with customer preferences. By understanding the attributes that consumers value most, companies can prioritise these in their product development and marketing strategies. This approach ensures that the features of a product are not just appealing in theory but are also what drive consumer decisions in practice.
For instance, a smartphone manufacturer might use DCM to determine which features (camera quality, battery life, screen size, etc.) are most valued by their target customers. This information can guide the manufacturer in allocating resources more efficiently, focusing on enhancing these key features rather than investing in the less influential aspects of consumer choice.
Steps to Conduct a Discrete Choice Modelling (DCM) Study
Let’s introduce a low-sugar cereal brand called “Wholesome Crunch.” The brand is known for its commitment to healthy eating and wants to expand its market share. Conducting a Discrete Choice Modelling (DCM) study for a low-sugar cereal brand involves several key steps. This type of study helps understand consumer preferences and the trade-offs they are willing to make when selecting cereal products. Here’s how the study can be structured, including the consideration of alternatives:
Step 1: Define the Research Objective for Wholesome Crunch’s DCM Study:
The first step in a DCM study is clearly defining the research objective. This involves specifying what the study aims to understand or predict about consumer behaviour. Objectives can range from determining the most valued product features to understanding price sensitivity to segmenting the market based on preferences. A well-defined objective guides the entire process, ensuring the study stays focused and relevant.
In our example, the primary objective of Wholesome Crunch’s DCM study is to identify critical factors that influence consumer decisions when choosing low-sugar cereals. Specifically, the study aims to:
- Understand Consumer Preferences: Determine which attributes of low-sugar cereals are most valued by consumers. This could include taste, nutritional content, price, packaging, and brand reputation.
- Evaluate Price Sensitivity: Assess consumers’ sensitivity to the price of low-sugar cereals. This will help Wholesome Crunch understand the optimal pricing strategy for their products.
- Identify Market Segments: Segment the market based on consumer preferences and behaviours. This could involve categorising consumers who prioritise health benefits over price or prefer certain flavours.
- Optimise Product Features: Use the insights gained to refine existing products or develop new ones that align more closely with consumer preferences.
- Test Market Reactions to New Ideas: Evaluate how the target market might receive potential changes, such as introducing a new flavour or altering nutritional content.
By clearly defining these objectives, Wholesome Crunch ensures that their DCM study is tailored to provide actionable insights that can directly impact their marketing strategies and product development. This focused approach will enable them to meet their consumers’ needs better and strengthen their position in the competitive low-sugar cereal market.
Step 2: Design the Choice Experiment
The second step involves designing the choice experiment. This is a critical phase where hypothetical cereal products are presented to respondents, who are then asked to choose between them based on varying attributes. In our example, here’s how Wholesome Crunch would proceed:
Selection of Attributes:
Wholesome Crunch selects key attributes for their cereals based on the research objectives. These might include:
- Sugar Content (e.g., no added sugar, low sugar)
- Price (e.g., budget-friendly, mid-range, premium)
- Flavour (e.g., plain, honey, chocolate, fruit)
- Packaging (e.g., standard, family-size, eco-friendly)
- Nutritional Additives (e.g., high fibre, added vitamins, protein-enriched)
For each attribute, different levels are defined to represent the range of options available in the market. For example:
- Sugar Content: 0g, 2g, 5g per serving
- Price: $3.99, $4.99, $5.99
- Flavour: Plain, Honey, Chocolate, Fruit
- Packaging: 250g standard box, 500g family box, 250g eco-friendly packaging
- Nutritional Additives: None, High Fiber, Vitamin-Enriched, Protein-Enriched
Various cereal product profiles are created using these attributes and levels. Each profile represents a different combination, akin to actual cereal products on the shelves.
For instance, one profile might be a low-sugar (2g), mid-range priced ($4.99), honey-flavoured cereal in eco-friendly packaging with high fibre content.
Designing Choice Sets:
Respondents are presented with sets of these hypothetical cereal products.
Each set includes a few different cereal options (typically 3-5) and an option to choose none.
The sets are designed to ensure that respondents face trade-offs, mirroring real-world decision-making.
Incorporating Realism and Relevance:
The scenarios are crafted to be as realistic as possible, reflecting consumers’ choices in stores.
Step 3: Survey Development:
Develop a questionnaire including the choice sets. Include demographic and lifestyle questions to segment the responses.
Step 4: Collecting and Analysing Data
Sampling and Data Collection:
Define your target audience (e.g., health-conscious consumers and families), and collect data through surveys distributed to a representative sample of your target market.
Modelling and Data Analysis:
Use statistical software to run the DCM analysis. Analyse how different attributes and their levels influence consumer choices and calculate the relative importance of each attribute in the decision-making process.
Including Alternatives:
Ensure the alternatives in each choice set reflect real market options. Include competitor brands and different variants of low-sugar cereals. Even consider including a high-sugar cereal option for this analysis. With the experiment designed, the next phase is data collection. This typically involves surveying a representative sample of the target market. Respondents are presented with the choice sets, and their selections are recorded. The collected data is then analysed using statistical models. This analysis aims to quantify the relationship between the attributes of the alternatives and the choices made by the respondents. This analysis often involves complex statistical software and requires expertise in statistics and consumer behaviour.
Step 5: Drawing Actionable Insights
The final step is to interpret the results of the data analysis and draw actionable insights. This involves translating the statistical findings into meaningful business insights. For instance, if the study reveals that price is less significant than brand reputation in consumer choice, this insight can guide pricing and branding strategies for Wholesome Crunch. The key is to transform the data-driven insights into practical, actionable strategies that align with the business’s overall goals.
Challenges and Limitations of Discrete Choice Modelling (DCM)
Potential Pitfalls in DCM Studies
DCM is a powerful tool, but like any method, it comes with challenges and limitations that can affect the validity of its results.
Complexity of Real-World Choices: One of the main challenges is the complexity of real-world decision-making processes. Consumers often make choices based on a combination of rational analysis and emotional or subconscious factors that can be difficult to capture in a model.
Over-Simplification of Choices: There is a risk of oversimplifying the choices in the experiment design. If the choice sets don’t accurately reflect the complexity and nuances of real-world scenarios, the results may not be applicable or useful.
Survey Bias: The way a survey is conducted can introduce biases. Questionnaire design, the context in which questions are asked, and the sample of respondents chosen for the study can all impact the accuracy of the data.
Handling of Unobserved Variables: Not all factors influencing choice can be observed or measured. Ignoring these unobserved variables can lead to incorrect conclusions.
Model Assumptions: DCM relies on certain assumptions, like the independence of irrelevant alternatives. The model’s predictions can be skewed if these assumptions don’t hold true in the real world.
Overcoming Common Challenges
In-Depth Understanding of the Market: To address the complexity of real-world choices, researchers should deeply understand the market and the consumers they are studying. This understanding helps in designing more realistic choice scenarios.
Robust Experiment Design: The careful design of the choice experiment is crucial. This includes ensuring that the attributes included are comprehensive and represent real trade-offs that consumers face.
Mitigating Survey Bias: To counter survey bias, it’s essential to use well-designed questionnaires and ensure that the sample of respondents represents the broader market.
Incorporating Advanced Techniques: Advanced statistical techniques, like mixed logit models, can help deal with unobserved variables and relax some of the rigid assumptions of traditional models.
Continuous Testing and Validation: Regularly testing and validating the model against actual market data can help ensure that the model remains accurate and relevant.
Emerging Trends and Technological Advancements in Discrete Choice Modelling (DCM)
Discrete Choice Modelling is rapidly evolving, driven by significant technological advancements. These developments enhance DCM’s accuracy, efficiency, and applicability.
Integration with Big Data: The explosion of big data has provided a wealth of information that can be integrated into DCM studies. Big data sources, like social media, consumer behaviour data, and IoT (Internet of Things) devices, offer real-time and more granular insights into consumer preferences and behaviours.
Advanced Analytics and Machine Learning: Incorporating machine learning algorithms and advanced analytics into DCM revolutionises data analysis. These technologies allow for handling larger datasets and more complex models, leading to more nuanced and accurate predictions.
Improved Survey Techniques: Technological advancements in survey methods, including mobile and web-based surveys, make data collection more efficient and far-reaching. This has expanded the scope and scale of DCM studies, allowing for a more diverse and representative sample of respondents.
The Future of DCM in the Age of Big Data and Machine Learning
Looking forward, the integration of DCM with big data and machine learning is set to redefine the field.
Predictive Analytics: The future of DCM lies in predictive analytics, where machine learning models can analyse vast datasets to predict future consumer behaviour patterns. This approach will move DCM from explaining past choices to forecasting future consumer decisions.
Personalisation at Scale: As machine learning models become more sophisticated, they will enable businesses to understand and predict individual consumer preferences at scale. This will open up new possibilities for personalised marketing and product development.
Dynamic Modelling: Combining DCM with real-time data streams will lead to dynamic models that can adapt and evolve as consumer preferences change. This will be crucial in fast-paced markets where trends and consumer behaviours shift rapidly.
Ethical and Privacy Considerations: With the increasing use of personal data, ethical and privacy considerations will become more prominent. Ensuring data security and privacy will be vital in maintaining consumer trust.
Cross-Disciplinary Applications: The applications of DCM will extend beyond traditional marketing and economics, potentially influencing fields like urban planning, healthcare, and environmental policy, where understanding human choices is crucial.
Final Thoughts: The Significance of Discrete Choice Modelling
Discrete Choice Modelling (DCM) stands at the forefront of decoding consumer behaviour, a vital compass in navigating the intricacy of market preferences. It offers a lens through which the nuances of consumer decisions become visible and quantifiable. As we have explored, DCM delves deep into understanding the ‘why’ behind consumer choices, bringing to light the subtle interplay of preferences and trade-offs that guide purchasing decisions.
As markets become saturated and consumer attention fragmented, DCM helps brands align their offerings with the ever-evolving desires of their customers. It goes beyond traditional market research, providing a dynamic framework that adapts to the complexities of human choice. The insights from DCM empower brands to tailor their products, services, and marketing strategies with unprecedented precision, turning guesswork into strategic decision-making.
Integrating technological advancements such as big data analytics and machine learning propels DCM into new possibilities. This evolution positions DCM as a tool for understanding current market dynamics and a predictive engine that can anticipate future trends and consumer behaviours.
However, the ethical implications of harnessing vast amounts of consumer data and its privacy concerns cannot be overlooked. As DCM continues to evolve, it must be keenly aware of these issues, ensuring consumer trust is not compromised in pursuing market insights.
Discrete Choice Modelling is a strategic ally in the quest to understand and cater to the multifaceted nature of consumer preferences. Its significance in guiding brands towards more consumer-centric approaches is undeniable. As we progress, DCM will undoubtedly play a pivotal role in shaping market strategies, driving innovation, and fostering a deeper connection between businesses and their customers.
Get regular insights
Keep up to date with the latest insights from our research as well as all our company news in our free monthly newsletter.
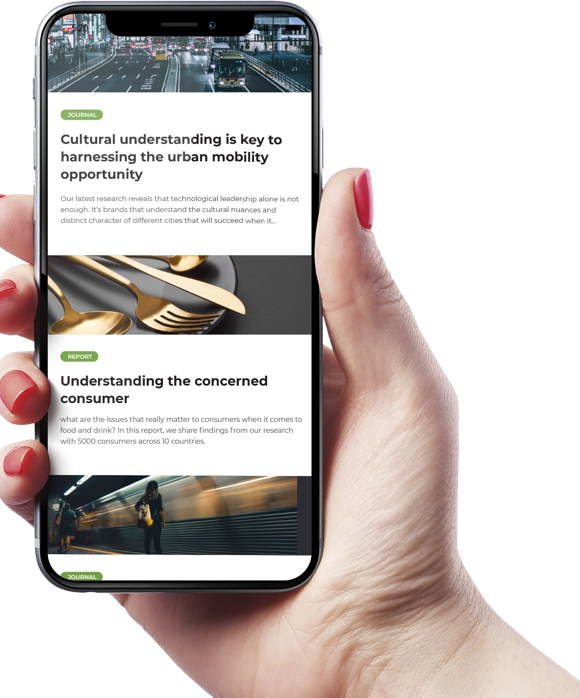