In market research, the sands are constantly shifting beneath our feet. Just when you think you’ve got a grip on the latest trend or technology, another wave of innovation comes crashing in, promising to revolutionise the industry. Remember when online surveys were all the rage? Or the influx of big data analytics that we thought would be the answer to all our research queries? Today, there’s a new buzzword on everyone’s lips: synthetic data.
Imagine having a dataset that looks and feels like your target market but doesn’t involve prying into anyone’s personal life. That’s the magic of synthetic data. Synthetic data is crafted through algorithms and models to mimic the structure and patterns of actual data without the baggage of privacy concerns or accessibility challenges.
But like all tools in our arsenal, synthetic data isn’t without its critics or challenges. While it has the potential to usher in a new era of flexible, privacy-compliant research, it’s essential to understand its role in the broader data landscape. The question is: Is synthetic data the future of market research, or just another tool in our ever-expanding toolbox?
The State of the Industry
Let’s journey back to when synthetic data was in its infancy. While today it’s making waves in our industry, it wasn’t too long ago when synthetic data was a mere whisper among data scientists. Its roots trace back to fields outside of market research – primarily in sectors like healthcare and finance, where the challenge was twofold: harnessing vast amounts of data while ensuring utmost privacy. And so, synthetic data was born out of necessity, a solution to simulate real-world data free from the constraints of sensitive information.
Fast forward to the present day, when the market research industry is facing its own set of unique challenges. With an increasingly globalised world and a maze of data privacy laws, market researchers have been searching for innovative ways to navigate this tricky landscape. Enter synthetic data, offering a promise of large-scale, representative datasets without the accompanying legal and ethical baggage.
According to MarketsandMarkets, the global synthetic data generation market will grow from USD 0.3 billion in 2023 to USD 2.1 billion by 2028.
Synthetic data, it seems, isn’t just knocking on the door of market research—it’s already set foot in the room.
Unpacking Synthetic Data
At this juncture, we must demystify what synthetic data truly is. In an industry awash with jargon and buzzwords, it’s easy to lose sight of the essence of a term, and “synthetic data” is no exception. So, let’s break it down.
Imagine an artist who’s never seen an actual sunset but has read about its colours, its patterns, and emotions it evokes. Using this information, they paint a sunset. While it’s not a reflection of an actual sunset they’ve witnessed, it captures the essence, the characteristics, and the general feel of one. This is the essence of synthetic data. It’s data that hasn’t been directly observed or collected from real-world events but has been algorithmically crafted to resemble and mimic real data in its structure, patterns, and behaviour.
Synthetic data is birthed through advanced computational models and algorithms. By feeding these models with existing real-world data, they learn its intricate nuances, patterns, and correlations. And, like a skilled artist, these models generate new data that, while not real, aligns closely with the patterns of the original. In the best cases, this generated data becomes almost indistinguishable from genuine data, mirroring the intricacies of our real-world observations.
But why does this matter to the market researcher? Because, in essence, synthetic data offers a powerful proxy. It provides a canvas to test hypotheses, model scenarios, and glean insights in environments where using real data might be cumbersome, ethically challenging, or downright impossible. It’s a tool, and like all tools, its efficacy lies in how adeptly we wield it.
Key Use Cases in Market Research
Scenario Testing and Simulations: Picture this: You’re about to launch a new product with high stakes. Traditional methods might offer insights based on past trends and data, but what if you could simulate a plethora of possible future scenarios to gauge potential outcomes?
With synthetic data, you can. It allows researchers to create hypothetical markets, consumer reactions, and competitive responses, offering a sandbox environment to test strategies and anticipate challenges.
Model Training and Validation: Machine learning models and AI-driven analytics are only as good as the data they’re trained on. But amassing vast, diverse, and representative datasets is a tall order. Enter synthetic data. Researchers can train more robust, accurate, and resilient models by bolstering real-world datasets with synthetic counterparts.
Furthermore, using synthetic data for validation ensures that the model’s insights and predictions align with varied scenarios, not just the limited scope of original datasets.
Data Augmentation: Sometimes, the real-world data we possess is patchy, sparse, or glaringly imbalanced. For instance, consider a study where responses from a particular demographic are underrepresented. Rather than restarting the data collection process—a daunting and costly endeavour—synthetic data can fill these gaps. Researchers can achieve a more holistic, balanced view of the market landscape by generating data that mirrors the missing or underrepresented segments.
Privacy-Compliant Research: The global shift towards stricter data protection regulations—think GDPR in Europe or CCPA in California—has thrown many researchers into a conundrum. How does one extract deep insights while staying within the bounds of these stringent laws? Synthetic data offers a beacon of hope. Since it doesn’t originate from real individuals but is algorithmically generated, it sidesteps the personal data pitfalls. Researchers can thus delve deep into data analytics without the looming cloud of privacy breaches.
The Allure: Benefits of Synthetic Data
The allure of synthetic data isn’t just in its novelty. It lies in its profound potential to transform the way we approach market research, offering solutions that are in tune with our industry’s modern challenges and aspirations.
Addressing Privacy and Data Access Concerns: With global consumers becoming increasingly privacy-conscious and data breaches making headlines, the ethical handling of data has never been more critical. Synthetic data elegantly sidesteps these concerns. As it’s derived from algorithms and not direct individual records, it offers a way to conduct comprehensive research devoid of personal data complications. Thus, it ensures that our pursuit of insights doesn’t come at the cost of individual privacy.
Potential Cost and Time Efficiencies: Traditional data collection methods, be it surveys, focus groups, or observational studies, can be time-consuming and heavy on the pocket. Generating synthetic data, once the initial models are set up, can be considerably faster and more cost-effective. Instead of repeated data collection efforts, researchers can generate fresh data on demand, leading to quicker turnarounds and potentially reduced project costs.
Flexibility and Scalability in Research Design: Imagine being able to tweak your dataset in real time to cater to evolving research questions or to simulate different market scenarios. Synthetic data offers this dynamism. Whether you need to upscale the dataset to represent a larger audience or adjust parameters for a new demographic, synthetic data provides an adaptability that’s hard to achieve with traditional datasets.
Enhancing and Enriching Datasets for Deeper Insights: Often, our datasets, while rich, might have gaps or areas of shallowness. Instead of returning to the drawing board, synthetic data allows for augmentation. By filling in the gaps or adding depth where needed, it ensures that our analyses are well-rounded. The result? Insights that are more comprehensive, nuanced, and reflective of the complexities of the market.
The Flip Side: Limitations and Concerns
Every silver lining has its own cloud, and there are undeniably some shadows in synthetic data. While its benefits are transformative, it’s paramount for market researchers to be aware of the potential pitfalls that accompany this data revolution.
Quality and Representativeness Issues: Synthetic data is a reflection, an echo of the real thing. And like any reflection, it can sometimes be distorted. The effectiveness of synthetic data hinges on how accurately it captures the nuances of real-world data. The derived insights risk being superficial or misleading if they fail to mirror the intricate patterns and structures. The challenge? Ensuring that this artificial construct truly epitomises the complexities of genuine datasets.
Potential Propagation of Biases: Synthetic data, for all its algorithmic brilliance, is still a child of its parent data. If the original dataset carries subtle or glaring biases, the synthetic offspring will likely inherit and potentially amplify them. For instance, if historical data is skewed towards a particular demographic due to past oversights, the synthetic data will mirror this skewness, leading to conclusions that perpetuate these biases.
Overfitting Risks in Machine Learning Models: Machine learning model’s prowess is often tested by its ability to generalise, to perform well on unseen data. Training models on synthetic data run the risk of overfitting, where the model becomes too attuned to the synthetic dataset’s quirks. While it might boast impressive performance metrics on the synthetic data, it could falter when faced with real-world scenarios.
Ethical Considerations and the Risk of Misinterpretation: Just because we can generate synthetic data, does it always mean we should? The line between genuine insights and data manipulation can sometimes blur. There’s also the danger of stakeholders misinterpreting or overvaluing insights derived solely from synthetic data, leading to decisions that might not stand the test of real-world unpredictabilities.
Brands and Synthetic Data: Why Make the Shift?
Brands constantly seek that elusive edge, the differentiator that propels them ahead of the curve. In this pursuit, data has always been a trusted ally. But with the emergence of synthetic data, the question beckons: Why should brands shift gears?
Cost Efficiency: For brands, every decision is, at its core, an ROI calculation. Traditional research, while invaluable, often comes with significant costs – both in terms of money and time. Synthetic data, with its ability to be generated on-demand, offers brands a more cost-effective avenue. Instead of recurrent expenditures on fresh data collection, synthetic data provides continuous insights without consistently draining resources.
Agility in Research: Brands that can pivot, adapt, and respond with agility are the ones that thrive. With its dynamic nature, synthetic data empowers brands to modify research parameters on the fly, test new hypotheses swiftly, and get answers without the wait times typical of conventional research methods.
Compliance with Data Regulations: In an era where data privacy regulations are tightening their grip globally, brands are walking a tightrope. How does one delve deep into consumer insights without running afoul of these regulations? Synthetic data offers a lifeline. By leveraging data that mirrors real-world patterns without stemming from individual personal records, brands can sidestep potential regulatory landmines, ensuring their research is insightful and compliant.
Competitive Edge with Richer Datasets: Having a richer dataset is akin to wielding a sharper sword. Synthetic data allows brands to augment their existing data reservoirs, leading to deeper, more nuanced insights. This depth can be the difference between a generic strategy and a bespoke solution, giving brands a distinct competitive advantage.
Strategic Advantage of Scenario Simulations: Uncertainty is the only certainty in today’s markets. With factors like global events, shifting consumer behaviours, and disruptive innovations, brands are often in uncharted waters. Synthetic data offers a compass. By simulating various market scenarios, from the optimistic to the catastrophic, brands can strategise with foresight, preparing for a spectrum of possibilities rather than being blindsided.
Real-world Pitfalls: When Synthetic Data Falls Short
While the allure of synthetic data is undeniable, it’s crucial to approach its integration with a discerning eye. In the real-world application of any pioneering technology, there are bound to be missteps and miscalculations. For all its promise, synthetic data has had its share of pitfalls.
Flawed Applications
- Biases in Hiring Algorithms: Consider the tech industry’s endeavour to automate the recruitment process using AI. By relying on synthetic data generated from historical hiring patterns, some firms inadvertently codified existing biases. The result? Algorithms that favoured specific demographics over others, perpetuating and amplifying historical imbalances rather than rectifying them.
- Misrepresentation in Consumer Preferences: In e-commerce, synthetic data was once used to predict emerging consumer trends. But without a robust foundation in genuine consumer behaviours, the resultant predictions skewed towards past patterns, missing out on evolving tastes and shifts in preferences. Brands relying solely on these insights found themselves misaligned with the market pulse.
Consequences of Over-reliance
- Lack of Grounded Insights: Synthetic data, while a potent tool, is a reflection, not the reality. Over-reliance without validation can lead to insights that, while mathematically sound, lack grounding in real-world nuances. This disconnection can result in strategies that are theoretically optimal but practically ineffectual.
- Overfitting in Predictive Models: Training models predominantly on synthetic data can be a double-edged sword for brands venturing into predictive analytics using machine learning. Such models exhibit stellar performance metrics on synthetic datasets but falter in real-world applications, leading to off-mark predictions or strategies that miss their target.
- Ethical and Reputational Hazards: Missteps in synthetic data application, especially when biases are amplified, can lead to strategic errors and ethical quandaries. The reputational damage from perceived insensitivity or discrimination can be long-lasting, undermining brand trust and equity.
Charting the Synthetic Horizon: Navigating with Purpose
With its myriad capabilities, synthetic data beckons us toward new methodologies, richer insights, and more efficient processes. But it’s crucial to recognise it for what it is: a formidable tool, not the final destination.
While synthetic data heralds a new dawn for market research, it’s not without its twilight zones. It demands of us a balance of enthusiasm and caution, a keen understanding of its strengths and weaknesses, and an unwavering commitment to ethical research practices. After all, in our quest for deeper insights, we must ensure that the compass of integrity and accuracy remains our steadfast guide.
The essence of market research, the heart of our profession, lies in understanding, unveiling truths, and deciphering the myriad complexities of human behaviour and market dynamics. Synthetic data can aid, guide, and even elevate our pursuits. But it cannot—and should not—become a replacement for the core tenets of diligent research and genuine human insights.
Get regular insights
Keep up to date with the latest insights from our research as well as all our company news in our free monthly newsletter.
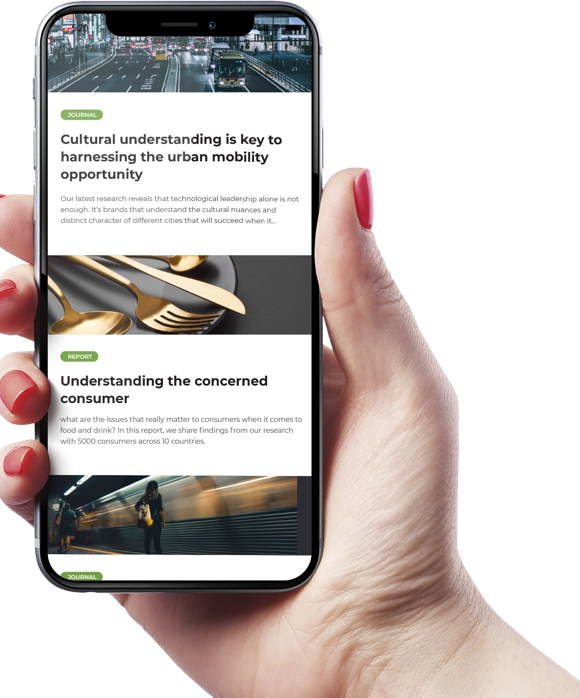