Market research is more than a routine step in a business plan; it’s the compass steering companies through shifting consumer behaviors, emerging trends, and competitive pressures. Think of it as both a map outlining the broader landscape and a magnifying glass revealing the subtle details that influence strategic decisions. Whether in the brainstorming sessions of startups or the boardrooms of Fortune 500 giants, market research grounds business strategies in evidence, not assumptions.
Beyond spreadsheets and statistics, market research is, at its core, about storytelling – uncovering the voice of the customer and translating it into insights that drive action. In a world shaped by rapid technological shifts, evolving consumer expectations, and relentless global competition, understanding market dynamics isn’t a luxury – it’s the difference between growth and stagnation.
Market research goes beyond identifying the ‘what’; it uncovers the ‘why’ behind consumer behavior. It’s the critical link between raw data and strategic decisions, shaping everything from product development to marketing campaigns and growth strategies. When done well, it doesn’t just inform – it reveals untapped opportunities, reduces risks, and gives businesses the clarity to stay ahead in crowded markets.
Types of Market Research
Market research takes many forms, each crafted to uncover insights tailored to distinct business challenges. Its varied approaches mirror the complexity of today’s markets, where no single method can capture the full picture. From foundational studies to advanced data analytics, every technique adds a vital piece to the puzzle of understanding market dynamics.
Primary Research: Primary research is the investigative backbone of market research – where businesses go straight to the source to gather firsthand insights. Through surveys, interviews, focus groups, and observations, companies can uncover not just what consumers think, but why they feel that way. Picture a tech startup rolling out a new app: beyond measuring user satisfaction, interviews can expose hidden frustrations, shaping more intuitive design updates. This direct, hands-on approach delivers raw, unfiltered data, giving businesses an edge rooted in real consumer experiences.
Consider a fictional case of a global beverage brand aiming to break into a new market. Through street interviews and interactive focus groups, the company uncovers that local consumers favor unique flavor profiles, diverging sharply from its standard offerings. Equipped with these insights, the brand launches a new product line tailored to regional tastes, outperforming competitors who relied solely on secondary data.
Secondary Research: While primary research gathers data firsthand, secondary research is more like investigative journalism – synthesizing insights from existing sources. This approach taps into industry reports, academic studies, government data, and competitor analyses to build a comprehensive picture. Imagine a company exploring entry into a new international market: by examining consumer spending trends, regulatory landscapes, and competitor strategies, they can benchmark their approach effectively. It’s a cost-efficient, time-saving method that’s indispensable for broad market assessments.
The strength of secondary research lies in its ability to provide context. For example, a global retailer expanding into emerging markets might rely on government economic reports, industry analyses, and demographic data to refine its entry strategy. This data-driven approach helps businesses identify growth opportunities, anticipate challenges, and make informed decisions without starting from scratch.
Qualitative Research: Qualitative research dives deep into the human side of data, uncovering the emotions, motivations, and perceptions that drive behavior. Through methods like in-depth interviews, focus groups, and ethnographic studies, it reveals the underlying ‘why’ behind consumer decisions. Imagine a luxury fashion brand exploring perceptions of sustainability. A focus group might surface an unexpected contradiction: while consumers express strong support for eco-friendly practices, their purchasing choices often lean towards aesthetics and price. These nuanced insights help brands connect with their audiences in more authentic and meaningful ways.
Ethnographic studies push qualitative research even deeper by immersing researchers directly into the consumer’s environment. Rather than relying on secondhand accounts, researchers observe behaviors in real time, capturing authentic interactions and unspoken habits. For instance, by studying how people naturally engage with smart home devices in their own spaces, tech companies have uncovered hidden usability challenges – insights that have driven meaningful product design improvements to better reflect real-world usage.
Quantitative Research: If qualitative research uncovers the stories behind consumer behavior, quantitative research measures their scale and significance. It’s the science to qualitative’s art – relying on structured methods like surveys, experiments, and statistical analysis to transform behaviors, preferences, and trends into hard data. Consider an e-commerce platform analyzing thousands of transactions to detect seasonal purchasing patterns. These insights don’t just highlight trends; they provide the statistical confidence needed to inform large-scale decisions, from inventory management to marketing strategies.
Large-scale political surveys conducted ahead of elections are a striking example of quantitative research in action. By analyzing data from thousands of respondents, these surveys uncover voter trends and predict outcomes with impressive precision. Businesses apply similar data-driven techniques to forecast market shifts, measure brand health, and refine marketing strategies – turning raw numbers into actionable insights that shape competitive advantage.
Exploratory Research: Think of exploratory research as the scouting party of market research – designed to navigate uncharted territories where little is known. It’s particularly valuable for startups looking to uncover unmet needs or identify gaps in the market. Rather than seeking definitive answers, exploratory research thrives on discovery, helping businesses refine their hypotheses and chart the course for more targeted, in-depth studies.
Consider a hypothetical tech startup venturing into the wearable health device market with no prior industry experience. By conducting exploratory research – through informal interviews, brainstorming sessions, and competitor analysis – they uncovered a niche demand for senior-friendly devices with simplified features. This discovery didn’t just inform their thinking; it became the foundation for their product development and go-to-market strategy.
Descriptive Research: Descriptive research is the storyteller of data, capturing ‘what is’ by mapping out current market conditions, consumer demographics, and behavioral patterns. It doesn’t seek to explain why behaviors occur but focuses on painting an accurate picture of the present. For example, a consumer electronics brand might use descriptive research to analyze how different age groups engage with smart devices, generating insights that inform targeted product development and marketing strategies.
Take, for instance, streaming platforms that rely on descriptive analytics to track viewer habits. By analyzing which genres resonate with different age groups and regions, they can fine-tune content recommendations and develop original programming tailored to specific audience segments. This data-driven approach helps platforms stay relevant in an increasingly competitive entertainment landscape.
When to Use Each Type of Market Research
Selecting the right research type is both an art and a science. It depends on the business objective at hand:
Objective | Recommended Research | Why It Works |
Understanding consumer motivations | Qualitative Research | Captures deep, emotional insights |
Measuring market size and trends | Quantitative Research | Provides statistically significant data |
Testing new product ideas | Exploratory Research, Focus Groups | Identifies potential opportunities |
Tracking brand awareness | Descriptive Research | Monitors changes and patterns |
Competitive analysis | Secondary Research | Leverages existing data efficiently |
Direct customer feedback | Primary Research (Surveys, Interviews) | Offers real-time, specific insights |
Blending these methods often yields the richest insights, as each type compensates for the limitations of the others.
Sharpening Your Market Research Edge
Markets don’t sit still – and neither should the research that guides business decisions. As consumer habits shift with every technological leap, cultural trend, or global disruption, the methods companies use to understand their audiences must evolve in real time. Market research isn’t a static report filed away after a product launch; it’s a living process, one that sharpens with every new question, data point, and insight. The businesses that thrive aren’t just collecting data – they’re continuously refining how they gather, interpret, and act on it.
Define Clear Objectives
Good research starts with a good question. The difference between insightful data and a meaningless spreadsheet often comes down to how clearly the objective is defined. Broad, unfocused goals – like ‘What do our customers think?’ – rarely produce actionable insights. Instead, sharp, specific questions drive meaningful outcomes. For example, rather than asking, ‘How do people feel about our brand?’ a company might ask, ‘What motivates Gen Z consumers to choose our product over competitors?’ or ‘Which factors most influence brand loyalty among urban millennials?’ These targeted inquiries not only shape the research process but also ensure that the answers can directly inform business decisions.
Blend Data Sources
Relying on a single source of data is like trying to solve a puzzle with half the pieces missing. The most valuable insights come from stitching together information from multiple angles. Primary research – surveys, interviews, focus groups – offers firsthand perspectives straight from the target audience. But layering in secondary data, such as industry reports, competitor analysis, and even social media trends, provides context that raw numbers alone can’t capture. A company exploring international expansion might combine customer interviews in new markets with economic data, competitor case studies, and social listening tools to paint a complete picture. This blend of sources helps validate findings, uncover hidden patterns, and reduce the risk of bias.
Leverage Technology
Tech hasn’t just changed what we research – it’s revolutionized how we do it. Gone are the days when gathering consumer insights meant months of manual data collection. Today, AI-driven analytics can process vast datasets in seconds, identifying patterns invisible to the human eye. Sentiment analysis tools track how customers feel about brands in real time, while dynamic dashboards turn raw data into instantly digestible insights. For example, a retail brand can monitor the success of a marketing campaign as it unfolds, adjusting strategies based on real-time sales data and customer feedback. These tools don’t just speed up the process – they make it smarter, helping businesses pivot quickly in response to emerging trends.
Iterate and Adapt
Consumer behavior is a moving target. What resonates with an audience today might fall flat tomorrow, especially in industries driven by fast-changing trends. That’s why market research shouldn’t be treated as a one-and-done project. The most successful companies adopt an iterative approach, continually refining their strategies based on fresh data. Consider a streaming service that regularly tests new content recommendations, analyzing viewer engagement to tweak algorithms week by week. This agile mindset – where insights are constantly tested, updated, and applied – keeps businesses aligned with their audiences, even as preferences shift.
Foster Cross-Functional Collaboration
Market research isn’t just the domain of data analysts – it’s most powerful when it’s woven into every corner of an organization. Insights become more actionable when they’re shared across teams, from marketing and product development to sales and customer service. For instance, a product team might discover through user research that customers are confused by certain features. When that insight is shared with marketing, it can inform clearer messaging; when shared with sales, it can shape more effective pitches. Cross-functional collaboration ensures that research doesn’t just sit in a report – it drives decisions at every level.
From Data to Decisions
Collecting data is only half the battle. The real value lies in thoughtful analysis:
- Clean the Data: Ensure accuracy by removing inconsistencies.
- Code the Data: Organize qualitative responses for thematic analysis.
- Analyze: Apply statistical tools to uncover patterns.
- Interpret: Translate data into meaningful narratives that guide strategy.
- Report: Craft compelling reports with clear visuals to communicate findings effectively.
ROI of Market Research
Market research is often seen as an essential business function, but its true value lies in how effectively it drives results. In an era where every budget line is scrutinized, companies increasingly ask: What’s the return on investment (ROI) of our research efforts? The answer isn’t always straightforward because the impact of research extends beyond immediate revenue gains. It shapes strategies, reduces risks, and uncovers opportunities that might otherwise remain hidden. To quantify this value, businesses must look at both the tangible and intangible benefits. Here’s how to evaluate the ROI of market research meaningfully.
Set Clear Metrics
Measuring the ROI of market research starts with defining what success looks like. Vague goals lead to vague outcomes, making it difficult to link research efforts to business results. Instead of generic objectives like ‘gain customer insights,’ companies should set specific, measurable goals aligned with broader business strategies. For example, a company launching a new product might use research to reduce time-to-market by identifying the most promising features early on. Metrics could include increased sales, higher conversion rates, improved customer retention, or even reduced marketing spend due to more targeted campaigns. When the research objective is tied to a quantifiable outcome, it becomes much easier to demonstrate its value.
Cost-Benefit Analysis
At its core, ROI is about comparing what you spend to what you gain. But with market research, the equation isn’t always as simple as dollars in versus dollars out. The benefits often go beyond immediate revenue, encompassing factors like reduced product failure rates, optimized pricing strategies, and improved customer satisfaction. For instance, investing $50,000 in consumer research might seem steep – until it prevents a multi-million-dollar product flop by identifying a critical flaw before launch. Businesses should consider both direct returns, such as increased sales or lead generation, and indirect benefits, like more efficient resource allocation or enhanced brand positioning. This holistic view provides a more accurate picture of the research’s true value.
Track Long-Term Impact
While some research delivers quick wins, its greatest value often emerges over time. Market insights don’t just influence a single campaign or product – they shape long-term strategies, guide innovation, and build a deeper understanding of evolving consumer behavior. To capture this long-term impact, companies should track key performance indicators (KPIs) beyond the immediate aftermath of a research project. For example, a brand health study conducted today might reveal trends that influence product development decisions for years to come. Similarly, customer satisfaction research can lead to operational changes that improve retention rates over time. By regularly revisiting past research and assessing how its insights have informed business growth, companies can better quantify its enduring ROI.
Global Market Research Considerations
Expanding into global markets offers businesses access to new customer segments, diversified revenue streams, and growth opportunities. However, with these opportunities come layers of complexity that domestic research rarely encounters. Conducting market research across borders isn’t as simple as replicating a survey in multiple languages – it requires a deep understanding of cultural, regional, and logistical nuances. To gather insights that truly reflect diverse markets, businesses must adapt their research strategies to local contexts while maintaining a consistent global perspective. Here’s what to consider when navigating the complexities of global market research.”
Cultural Sensitivity
Culture shapes how people think, behave, and make decisions – making cultural sensitivity a cornerstone of effective global research. A question that resonates in one market might fall flat or even offend in another. For example, consumer attitudes toward money, health, or personal success vary widely across cultures, and even colors, symbols, or imagery used in surveys can carry different meanings. To avoid cultural missteps, businesses should collaborate with local researchers who understand these nuances. This goes beyond language translation; it’s about cultural adaptation. For instance, while Western consumers might be comfortable providing direct feedback, respondents in some Asian markets may prefer more indirect ways of expressing dissatisfaction. Recognizing these subtleties ensures the data collected is both respectful and reliable.
Regional Trends
Global doesn’t mean homogeneous. Consumer behaviors are shaped by regional trends influenced by local economies, political climates, technological adoption, and social norms. What drives purchasing decisions in North America might be entirely different from what resonates in Southeast Asia or the Middle East. Consider the rapid rise of mobile payments in China compared to the continued reliance on credit cards in the US. A one-size-fits-all research approach risks missing these critical distinctions. Businesses must stay attuned to local market dynamics – tracking everything from economic shifts and regulatory changes to emerging consumer preferences. Partnering with regional experts or leveraging localized data sources can provide a clearer picture of what matters most to consumers in specific markets.
Logistical Challenges
Conducting research across borders comes with practical hurdles that can affect both the quality and timeliness of insights. Language barriers are an obvious challenge, but even when surveys are accurately translated, subtle differences in phrasing can alter respondents’ interpretations. Time zone differences complicate scheduling interviews, focus groups, or real-time data collection efforts. Additionally, technological access varies widely – while online surveys may be standard in urban areas with high internet penetration, they might not reach rural populations in less connected regions. To overcome these obstacles, businesses should tailor their methodologies to fit the local context. This could mean combining digital surveys with face-to-face interviews, using mobile-based research in mobile-first economies, or ensuring data privacy compliance aligns with regional regulations like GDPR in Europe. Flexibility and local expertise are key to navigating these logistical complexities.
Emerging Trends in Market Research
The landscape of market research is evolving rapidly, driven by technological advancements and changing consumer behaviors. To stay competitive, businesses must adapt to these emerging trends that are reshaping how insights are gathered, analyzed, and applied.
- AI and Machine Learning:
Artificial Intelligence (AI) and Machine Learning (ML) are revolutionizing market research by automating data collection, enhancing predictive analytics, and uncovering patterns that traditional methods might miss. AI-powered tools can process vast datasets in real-time, enabling researchers to identify trends, forecast behaviors, and even segment audiences with precision. For example, sentiment analysis algorithms can scan millions of social media posts to gauge public perception of a brand instantly. This not only saves time but also provides more nuanced insights, helping businesses make data-driven decisions swiftly. - Social Listening:
In an era where consumers voice their opinions online, social listening has become indispensable. It goes beyond tracking brand mentions; it captures real-time consumer sentiment, identifies emerging trends, and monitors competitors. Platforms like Brandwatch and Sprinklr analyze data from social media, forums, and blogs, offering a comprehensive view of public discourse. For brands, this means staying ahead of potential PR crises, identifying new opportunities, and understanding customer needs without relying solely on traditional surveys. - Big Data Analytics:
The explosion of digital interactions has led to an abundance of data – commonly referred to as big data. Analyzing this data helps businesses uncover hidden patterns and correlations that inform strategic decisions. Big data analytics integrates information from various sources, such as transaction records, web analytics, and IoT devices, providing a holistic view of consumer behavior. Companies like Amazon and Netflix leverage big data to personalize recommendations, optimize supply chains, and predict market demands with remarkable accuracy. - Predictive Analytics:
While descriptive analytics tells us what happened, predictive analytics forecasts what’s likely to happen next. By using historical data, statistical algorithms, and machine learning techniques, businesses can anticipate future trends and consumer behaviors. This is particularly valuable in industries like retail and finance, where understanding purchasing patterns or market shifts can provide a competitive edge. Predictive models help in inventory management, targeted marketing campaigns, and even risk assessment. - The Rise of Agile Research:
Agile methodologies, borrowed from software development, are transforming market research. Agile research emphasizes speed, flexibility, and continuous feedback. Instead of lengthy, traditional research projects, agile approaches involve rapid cycles of data collection and analysis, allowing businesses to adapt quickly to changing market conditions. This is especially crucial in fast-paced industries where consumer preferences evolve rapidly, such as tech, fashion, and entertainment. - Emerging Technologies: Blockchain and AR/VR:
Emerging technologies like blockchain and augmented reality (AR) are also making inroads into market research. Blockchain ensures data transparency and security, addressing concerns about data integrity and privacy. Meanwhile, AR and virtual reality (VR) are being used for immersive research experiences, such as virtual focus groups or product testing in simulated environments. These technologies not only enhance data quality but also provide innovative ways to engage with participants.
In conclusion, the future of market research lies in the seamless integration of technology and human insight. As these trends continue to evolve, businesses that embrace and adapt to these innovations will be better positioned to understand their markets and anticipate changes.
Final Thoughts
Mastering market research is more than data collection; it’s about transforming insights into strategic action. In an era where consumer preferences shift rapidly, businesses that invest in robust, adaptive research methodologies position themselves for sustained success. Market research isn’t just a business function – it’s the strategic lens through which future opportunities come into focus.
Get regular insights
Keep up to date with the latest insights from our research as well as all our company news in our free monthly newsletter.
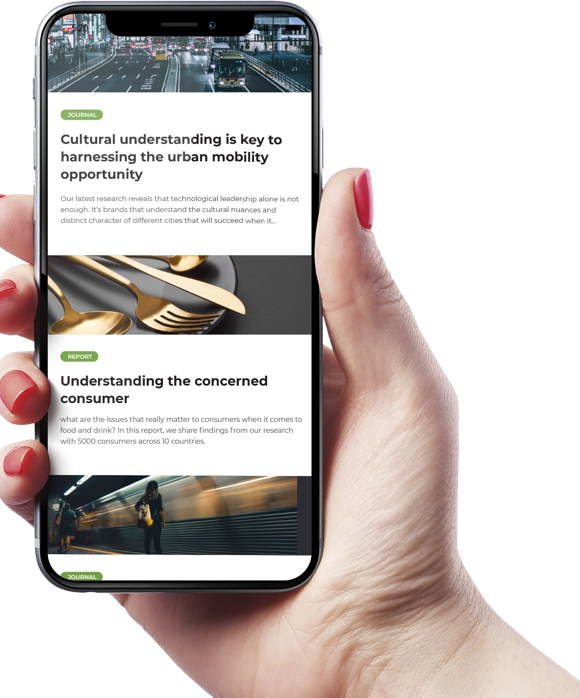