Today, every brand has a dashboard problem.
Real-time data tracks everything. Purchase paths are mapped down to the millisecond. Heatmaps show where consumers hover and hesitate. The real-time analytics market is booming, valued at $25 billion in 2023 and projected to reach $193.71 billion by 2032.
But in the race to be data-led, something is breaking.
Creativity gets boxed in by past behavior. Brand identity erodes under the weight of what’s trending. Short-term metrics win out over long-term vision.
Now, some of the world’s most ambitious brands are pushing back.
From fashion houses to fintech startups, companies are flipping the script — treating data not as a decision-maker but as a decision-support tool. This is data-informed leadership, where numbers sharpen instincts but never replace them.
Because the brands shaping the future aren’t the ones following the dashboard. They’re the ones willing to look up from it.
This is a real power move. This isn’t about ignoring data. It’s about knowing its place.
When Data Leads, Brands Lose Their Edge
Nowhere is the fallout of being data-led more visible than in marketing departments locked in endless loops of optimization.
Look at the wave of direct-to-consumer brands that flooded social feeds over the past decade. Fueled by performance marketing metrics – clickthrough rates, conversion percentages, cost-per-acquisition – these companies became masters of the micro-adjustment. Headlines were A/B tested to exhaustion. Product pages shifted based on heatmaps. Ads were churned out by the dozen, tweaked and re-tweaked until only the most clickable version survived.
Yet, many of these brands began to blur into one another – stripped of personality, chasing the same lookalike audiences with the same algorithm-friendly formulas.
Optimizing for KPIs without a clear brand compass is how brands lose their edge. The numbers might show what’s working now, but they rarely tell you whether anyone will care about your brand a year from now.
This is the risk brands face when they let data lead: it pulls them toward what’s proven, not what’s possible. It creates echo chambers of past behavior. And in a market where consumers crave identity, meaning, and human connection, it’s not enough to follow what the dashboard says.
Because the brands that are remembered – the ones people talk about, love, and come back to – don’t just follow patterns. They break them.
Data Can’t Read the Room
Data can tell you what people clicked. It can tell you how long they hovered over a product image. But it can’t tell you what made them laugh at the dinner table. It can’t decode why a slogan fell flat. And it certainly can’t predict the next cultural wave before it hits.
This is where market research proves its value – not as a report card on past behavior, but as a lens into the emerging culture, unmet needs, and emotional drivers that dashboards can’t track. Qualitative studies, ethnographic research, and in-depth interviews offer what raw analytics can’t: context, nuance, and human stories that decode the why behind the what.
Brands chasing data-led decisions often learn this the hard way. Take Pepsi’s 2017 advertisement featuring Kendall Jenner. The campaign aimed to resonate with younger audiences by aligning with themes of unity and protest – elements that data likely indicated were essential to this demographic. However, the execution was widely criticized for appearing to trivialize serious social justice movements, leading to public backlash and the eventual withdrawal of the ad.
This is where market research proves its value, not as a report card on past behavior, but as a lens into the emerging culture, unmet needs, and emotional drivers that dashboards can’t track. Qualitative studies, ethnographic research, and in-depth interviews offer what raw analytics can’t: context, nuance, and human stories that decode the why behind the what.
Where performance data might highlight rising engagement on social content using trending slang, a well-run focus group or semiotic analysis could reveal whether that language resonates, or risks alienating the audience by trying too hard. Research would have focused on tone, cultural sensitivity, and perceived authenticity long before backlash hit the feed. It doesn’t just show whether people noticed. It uncovers how they felt and why it matters.
Smart brands are starting to push back — treating data not as gospel but as one of many inputs in a much messier, more human process of understanding what matters to people.
This misstep underscores a critical limitation of data-led strategies: while analytics can highlight trends, they often lack the contextual understanding necessary to navigate complex cultural landscapes. Relying solely on data without human insight can result in messages that miss the mark, alienating the very audiences they intend to engage.
Smart brands are starting to push back – treating data not as gospel but as one of many inputs in a much messier, more human process of understanding what matters to people.
Data-Informed Brands Are Playing the Long Game
Across industries, a quiet shift is happening. The most resilient brands aren’t the ones chasing every data blip — they’re the ones brave enough to zoom out.
Consider Ben & Jerry’s, the iconic ice cream brand known for its bold flavors and unapologetic activism. In 2020, the company launched “Justice ReMix’d,” a limited-edition flavor supporting criminal justice reform. The campaign generated widespread buzz, and sales surged. Customers flooded social media and retail partners with requests to make the flavor permanent.
A data-led strategy might have given in. The metrics were strong, and the demand was obvious. But Ben & Jerry’s made a different call.
They chose to keep it a one-off, not because the numbers weren’t there, but because the long-term brand strategy was. The flavor wasn’t just a bestseller; it was a statement. Part of that impact came from its temporary nature—using ice cream as a cultural spotlight, not just a product.
This is what data-informed decision-making looks like: using numbers to gauge impact, but staying grounded in brand purpose. Sales figures and social metrics mattered, but didn’t override the strategic intent. Ben & Jerry’s understood the difference between what was popular now and what was authentic long term.
It’s the same muscle that other data-informed companies are flexing. They use data to pressure-test their instincts, to spark ideas, to avoid blind spots, but never to replace judgment. They know the difference between reacting and leading.
And in an era where consumers see right through opportunism, playing the long game isn’t just smart. It’s survival.
Case Study: Hugo Boss – Using Data to Guide Creativity, Not Replace It
Background
Hugo Boss, one of the world’s leading fashion brands, faced the same challenge confronting many legacy companies: how to embrace digital transformation without losing its creative edge. Under CEO Daniel Grieder, appointed in 2021, the company set an ambitious goal: to double sales to €4 billion by 2025.
Central to this ambition was a bold shift in strategy: becoming more data-driven while staying brand-led.
What the Hugo Boss Did
As part of its €150 million “Claim 5” strategy, Hugo Boss invested €15 million in a new Digital Campus in Gondomar, Portugal. This hub was designed to harness advanced data analytics across product design, marketing, and sales – creating faster feedback loops and operational efficiency.
But crucially, the company drew a line. Data was used to inform creative teams, not to dictate their decisions.
Designers still led product development based on brand vision and long-term strategy. Data helped validate ideas, identify emerging trends, and sharpen customer insights – but final decisions stayed rooted in brand instinct and creative direction.
Outcome
Hugo Boss’s approach has positioned it as a front-runner among heritage fashion brands navigating digital transformation. By resisting the trap of becoming purely data-led, the brand has maintained its distinct identity while accelerating growth.
Since implementing the strategy, Hugo Boss reported record sales in 2023 and is tracking ahead of its 2025 targets. Industry analysts have cited the company’s ability to blend creativity with smart data use as a key differentiator in a hyper-competitive market.
As CEO Daniel Grieder put it:
“Data can show us what’s happening – but creativity shows us what’s next.”
Product Teams Want Direction, Not Dictation
Inside product teams, the mood is shifting. For all the power of dashboards and predictive models, there’s growing fatigue with treating data like a final answer key.
Data shows you where the traffic is, not where the road should go. If teams only respond to what’s already happening, they’re not innovating; they’re following.
This is why product teams at some of the world’s most agile companies are moving away from absolutist, data-led roadmaps. Instead, they’re asking for directional data – insights that point to opportunity areas without shutting down creative thinking.
Increasingly, product development teams are leaning on market research not just to validate ideas but to shape them early – using consumer co-creation, concept testing, and journey mapping to pressure-test decisions before they go live. Research isn’t the finish line. It’s the starting point for informed creativity.
Consider Fisdom, a leading Indian fintech company. Through extensive UX research, Fisdom discovered that placing the Know Your Customer (KYC) form at the beginning of the signup process was a significant barrier for new users. By moving the KYC step to the end of the signup flow, they reduced friction and saw a notable increase in user completions. This change was guided not just by analytics but by a deeper understanding of user behavior and preferences.
This kind of data-informed decision-making is harder. It requires teams to accept ambiguity, weigh trade-offs, and trust their understanding of the customer beyond the numbers.
But it’s also where differentiation lives.
In the end, the smartest brands aren’t anti-data. They’re pro-human. They combine what the dashboard says with what market research uncovers – using evidence to sharpen their instincts, not replace them.
Data will always tell you what people did yesterday. Brand instinct decides what they’ll care about tomorrow.
Smart brands know the difference. The rest are just watching the dashboard.
At Kadence International, we help brands turn data into direction. As a global market research agency, we uncover the human insights behind the numbers, helping brands move with confidence, not (just) caution.
Get regular insights
Keep up to date with the latest insights from our research as well as all our company news in our free monthly newsletter.
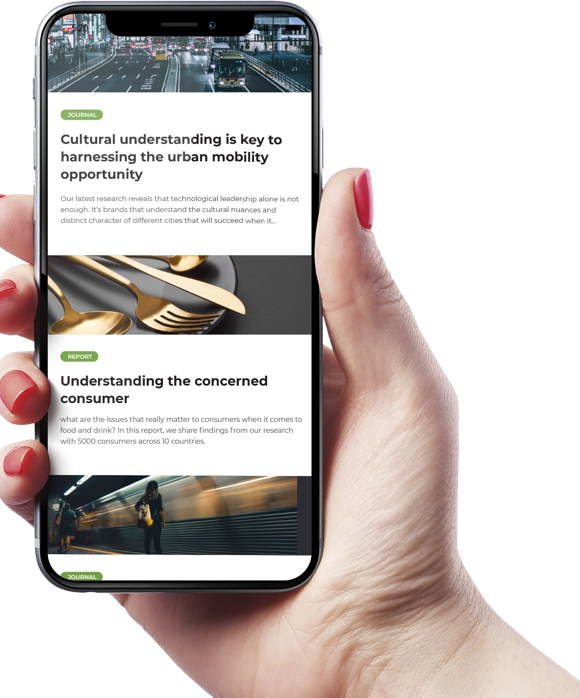