According to Statistica, by 2025, the global big data analytics market’s annual revenue will likely grow to $68.09 billion.
Data science is a beacon that guides corporations and individuals. It has revolutionized industries, from healthcare, where predictive algorithms can forecast outbreaks and patient needs, to finance, where it’s used to detect fraudulent transactions in real-time.
Data science, especially in the market research industry, is akin to discovering a new element —powerful yet not wholly understood. As its usage rises, a host of myths obscures its true nature.
Let’s examine the 12 common misconceptions about data science and learn the truth about this critical discipline.
Myth 1: Coding is the Sole Focus of Data Science.
The Reality: Yes, coding is a vital skill for data scientists. However, data science is a symphony, and coding is but one instrument. Other essential components include understanding business challenges, effective communication, and deep data comprehension.
Myth 2: Data Science, Machine Learning (ML), and Artificial Intelligence (AI) are Interchangeable Terms.
The Reality: While ML and AI are integral facets of data science, equating them is like saying a car is the same as its engine. Data science encompasses a broader spectrum, including traditional statistical analysis, data processing, and more.
“Without big data analytics, companies are blind and deaf, wandering out onto the web like deer on a freeway.” – Geoffrey Moore, Author and Consultant.
Myth 3: Data Science Guarantees Absolute Neutrality and Objectivity.
The Reality: Data might be neutral, but its collection, interpretation, and application can introduce biases. The objective is not to claim absolute neutrality but to continually strive for reduced bias and improved accuracy.
Myth 4: Data Science is Always a Walk in the Park.
The Reality: It’s a discipline that swings between eureka moments and bouts of intense, often tedious, work. Cleaning datasets or perfecting a model can be daunting. Yet, the rewards, both in insights and outcomes, can be immense.
“The goal is to turn data into information, and information into insight.” – Carly Fiorina, Former Executive, President, and Chair of Hewlett-Packard Co.
Myth 5: Data Science Offers Universal Solutions for Every Problem.
The Reality: Every organization has its unique nuances. While some data science approaches are widely applicable, they must often be tailored to fit specific business goals and challenges.
Myth 6: Data Science is Just a Fancy Term for Statistics.
The Reality: Statistics is a cornerstone, but data science transcends it. It’s about extracting insights from structured and unstructured data, not just number crunching.
Myth 7: More Data Equals More Accuracy.
The Reality: Quality trumps quantity. Mountains of irrelevant data can mislead, while a smaller, relevant dataset can offer profound insights.
Myth 8: Data Science Will Replace Traditional Market Research.
The Reality: Data science complements traditional research. It bridges quantitative insights and qualitative depth, offering a holistic understanding of the market landscape.
“Every day, we create 2.5 quintillion bytes of data.” (Marr, B. “How Much Data Do We Create Every Day? The Mind-Blowing Stats Everyone Should Read.”)
Myth 9: Only Large Corporations Need Data Science.
The Reality: Even small-scale businesses produce vast data in our interconnected world. From local bakeries analyzing customer preferences to startups gauging market fit, data science holds relevance across the board.
Myth 10: Data Scientists Work in Isolation.
The Reality: Collaboration is intrinsic to effective market research. Data scientists thrive when integrated with teams, blending their insights with broader business strategies.
Myth 11: Data Science Offers Instant Results.
The Reality: Some automated aspects may provide rapid insights, but profound analysis is a journey, not a sprint. Deep insights require time, patience, and refinement.
“The grunt work needed to clean complex data sets is critical to extracting real benefit, as insights are only as solid as the data they’re based upon,” Hassen Morad, Senior Data Visualization Analyst, Kadence International – Americas office.
Get the inside view into the world of data visualization in an interview with Hassen Morad, who leads our Americas data team. Read the full interview here.
Myth 12: All Data is Good Data.
The Reality: Indiscriminate data collection can lead astray. Each data point should have a clear purpose, serving the overarching research objective.
Data Scientists are not just number crunchers but storytellers, strategists, and innovators.
The role of a data scientist is multifaceted and continually evolving. Their day might begin with cleaning and preprocessing data to make it usable, then move to designing algorithms or building predictive models, and culminate in presenting findings to stakeholders in a comprehensible manner. All this requires more than just crunching numbers.
Here’s how data scientists helped solve a major market research problem for Airbnb’s vacation rental platform.
Airbnb’s Pricing Algorithm
The Challenge: When renting out a property on Airbnb, one of the most daunting tasks for hosts is deciding the price of their listing.
When priced too high, the property might be overlooked, and when priced too low, the hosts miss out on potential revenue. With a vast and varied inventory —from treehouses to penthouses in different locales, determining an optimal price is complex.
Airbnb realized that a significant percentage of their hosts were not pricing optimally. This impacted the hosts’ potential earnings and Airbnb’s revenue.
Enter Data Science.
Airbnb decided to assist hosts by developing a machine-learning model that suggests pricing tips based on a plethora of factors:
Historical Data: Analysing past booking data to understand pricing trends.
Temporal Trends: Considering seasonality, local events, and holidays which might impact demand.
Comparable Listings: Analyzing prices of similar listings in the vicinity.
User Behavior: Understanding how users interacted with the platform, their clicks, booking patterns, etc.
The Solution: The result was the “Smart Pricing” tool. Using this tool, hosts could let Airbnb set prices based on the model’s suggestions. The model continuously learns, adjusting prices as necessary. For instance, if a property wasn’t booked as expected, the model might suggest a price reduction. Conversely, if a particular locale saw increased demand because of a sudden event, the prices might be nudged higher.
Outcome: Airbnb hosts who adopted Smart Pricing saw a notable increase in their booking rates, ensuring that listings were priced competitively and advantageously. This not only improved the individual host’s earnings but also led to increased revenues for Airbnb as a platform.
The Future of Data Science
Data science is poised to break even more boundaries. Its potential applications are vast with the exponential growth of data generation and the advent of technologies like quantum computing and advanced neural networks. The rise of ethical considerations, such as bias in algorithms and data privacy, also means that the next generation of data scientists will operate at the intersection of technology and humanity.
The evolving narrative of market research is one of dynamic change, with data science as its most compelling protagonist. Yet, like any protagonist, it’s often misinterpreted, wrapped in layers of myth and misconception. For brands on the brink of innovation, understanding the real essence of data science can unlock transformative potential.
As we debunk these myths, we don’t just clarify; we illuminate the path to harnessing data’s genuine power, ensuring market research’s future is as luminous as its promise.
Get regular insights
Keep up to date with the latest insights from our research as well as all our company news in our free monthly newsletter.
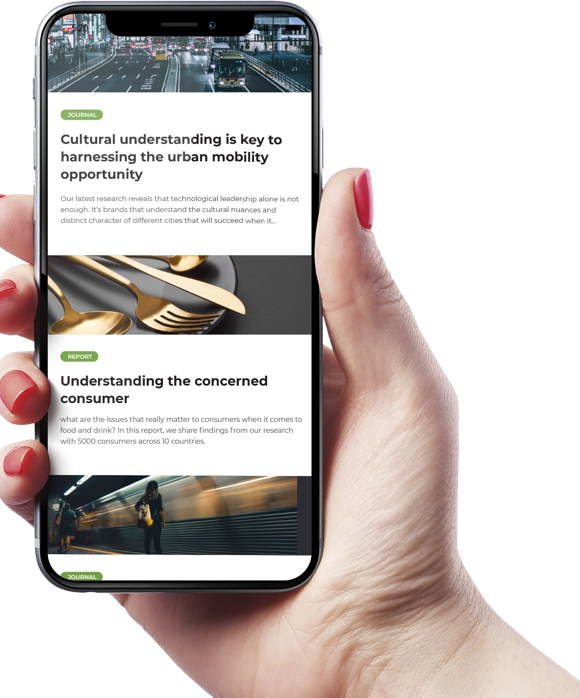