In today’s data-driven business landscape, finding the right balance between human judgment and machine analytics is crucial for making optimal decisions.
As more data becomes available and advanced analytics are refined, we face the challenge of determining when and how to incorporate automation while still leveraging our own expertise.
This article aims to delve into this critical topic, exploring the three common approaches to analytics (descriptive, predictive, and prescriptive) and addressing ethical considerations, data privacy, organisational change, industry case studies, and the importance of human-machine collaboration.
The Struggle for Balance
While machines excel in deduction, granularity, and scalability, humans possess unparalleled capabilities in intuition and ambiguity resolution. Determining the appropriate balance between the two is essential.
We often find ourselves grappling with questions such as when to shift from traditional human-centred methods to greater automation and how to strike a harmonious equilibrium between the two. To address these questions effectively, it is crucial to understand the three approaches to analytics and their applications.
Descriptive Analytics: Uncovering Insights from Historical Data
Descriptive analytics, often referred to as “business intelligence,” relies on machines to uncover patterns in historical data. It aims to answer the question, “Help me understand what happened.”
By using dashboards and aggregated information, we can make decisions based on verifiable and objective facts. However, descriptive analytics has limitations, including an overreliance on internal transaction data and a tendency to overlook external perspectives.
We can supplement this approach with our intuition and experience. It remains a valuable tool for providing directional guidance when data is limited, and uncertainty surrounds the outcome.
Predictive Analytics: Gaining Insights into Likely Outcomes
Predictive analytics involves machines determining likely outcomes based on different input variables. It helps answer the question, “What will happen?”
By leveraging forecasting models and analysing large datasets, we gain insights into potential future scenarios. However, predictive analytics faces challenges in accurately predicting complex interdependencies and incorporating all relevant factors influencing decisions. We can enhance predictive analytics by combining it with descriptive data and manual diagnostics. This approach is most suitable when there is more granular data available, decisions are frequent, and there are opportunities for quick wins.
Prescriptive Analytics: Harnessing Data for Granular Guidance
Prescriptive analytics empowers machines to make decisions based on defined objectives, leveraging large amounts of data to analyse market conditions. It answers the question, “What should I do now?”
This approach allows for rapid experimentation, automated optimisation, and continuous learning. While prescriptive analytics offers the potential for greater financial rewards and improved business performance, it requires dedicated software, hardware, and specialised expertise to set up effectively. The human role remains crucial in defining business rules and objectives, enabling machines to optimise outcomes while considering risk and economic costs.
Ethical Considerations: Ensuring Fairness and Transparency
As organisations adopt advanced analytics, ethical considerations come to the forefront. The potential biases present in data and algorithms necessitate careful attention to ensure fairness and equity in decision-making processes.
We must be proactive in identifying and mitigating biases, promoting transparency, and being accountable for the outcomes of automated decisions. Ethical considerations should encompass aspects such as algorithmic accountability, algorithmic fairness, and the ethical use of customer data.
Data Privacy and Security: Safeguarding Confidential Information
As the reliance on data grows, organisations must prioritise data privacy and security. Protecting sensitive information, complying with data regulations, and maintaining customer trust is essential.
We must implement robust data governance practices, establish secure data storage and transmission protocols, and continuously monitor and address emerging privacy and security risks. By prioritising data privacy and security, organisations can build trust with customers and stakeholders while mitigating potential legal and reputational consequences.
Organisational Change and Adoption: Navigating the Transition
Integrating advanced analytics approaches often requires significant organisational change. We must navigate the challenges of resistance to change, ensure alignment between analytics initiatives and strategic objectives, and foster a data-driven culture within the organisation.
This involves providing training and upskilling opportunities, encouraging collaboration between data scientists and business professionals, and establishing clear communication channels to address concerns and promote buy-in from all stakeholders.
Industry Case Studies: Illustrating Real-World Applications
Case Study 1: Financial Services – Fraud Detection
In the financial services industry, fraud detection is a critical concern. One case study involves a multinational bank that leveraged machine analytics to enhance its fraud detection capabilities.
By analysing large volumes of transactional data, customer behaviour patterns, and historical fraud incidents, the bank developed a predictive analytics model that flagged suspicious activities in real-time. The machine analytics system helped identify potentially fraudulent transactions with high accuracy, reducing false positives and enabling timely intervention by fraud detection teams.
This case study demonstrates the effectiveness of predictive analytics in improving fraud detection and safeguarding financial institutions and their customers.
Case Study 2: Healthcare – Patient Risk Assessment
In the healthcare sector, patient risk assessment plays a crucial role in optimising care and improving outcomes. One healthcare provider implemented prescriptive analytics to identify patients at a higher risk of readmission after discharge.
By analysing patient data, including medical history, lab results, and demographic information, the prescriptive analytics system generated risk scores for each patient. These risk scores guided care providers in designing personalised intervention plans, such as follow-up appointments, medication adjustments, and lifestyle recommendations.
The implementation of prescriptive analytics resulted in a significant reduction in readmission rates and improved patient outcomes. This case study showcases the power of prescriptive analytics in healthcare decision-making, enabling proactive interventions and resource allocation.
Case Study 3: Retail – Demand Forecasting
Retail organisations face challenges in accurately forecasting demand to optimise inventory management and avoid stockouts or overstocking. One retailer leveraged predictive analytics to improve demand forecasting and inventory optimisation.
By analysing historical sales data, customer behaviour, promotional activities, and external factors like seasonality and weather, the predictive analytics system generated accurate demand forecasts at both macro and micro levels. This allowed the retailer to optimise inventory levels, adjust pricing strategies, and plan promotions effectively.
As a result, the retailer experienced improved sales performance, reduced inventory costs, and enhanced customer satisfaction. This case study highlights the value of predictive analytics in retail decision-making, facilitating data-driven strategies for inventory management and revenue optimisation.
By examining these diverse case studies, we can gain insights into the real-world applications of analytics approaches in different industries. These examples demonstrate the benefits and challenges organisations encounter when leveraging human judgment and machine analytics, providing valuable lessons that can be adapted to our own specific contexts.
Human-Machine Collaboration: Harnessing Collective Intelligence
Recognising the strengths of both humans and machines, effective decision-making involves harnessing the power of collaboration. Humans bring intuition, contextual understanding, and creativity to the table, while machines excel in processing vast amounts of data and making repeatable decisions.
By integrating human judgment with machine insights, companies can achieve a synergistic effect, leveraging collective intelligence for better outcomes. Establishing feedback loops and communication channels between humans and machines fosters a dynamic and iterative decision-making process.
Final Thoughts
Finding the optimal balance between human judgment and machine analytics is a crucial endeavour for modern organisations. By understanding the three approaches to analytics, addressing ethical considerations, prioritising data privacy and security, navigating organisational change, exploring industry case studies, and fostering human-machine collaboration, we can make informed decisions that maximise the potential of both humans and machines.
Embracing this collaborative approach empowers companies to thrive in a data-driven world while ensuring ethical practices, safeguarding customer trust, and achieving superior business performance.
Get regular insights
Keep up to date with the latest insights from our research as well as all our company news in our free monthly newsletter.
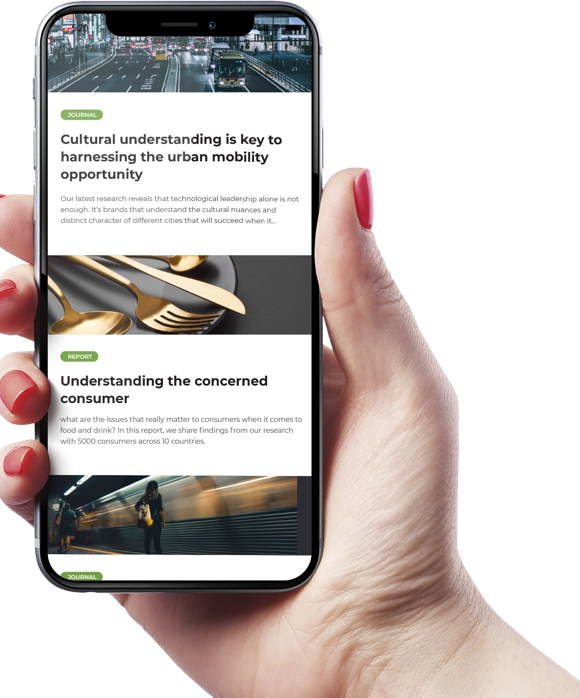