When OpenAI launched ChatGPT Pro, it positioned the $200-per-month premium subscription as an offering for power users. Yet, less than a year later, CEO Sam Altman revealed a surprising reality in a recent interview: the company is losing money on those subscriptions. “Insane thing: we are currently losing money on OpenAI Pro subscriptions! People use it much more than we expected,” Altman remarked.
This revelation underscores a critical oversight in one of the world’s fastest-growing tech companies. Despite OpenAI’s impact on artificial intelligence, its pricing strategy appears to have been driven by intuition rather than data. In the same interview, Altman admitted that the decision to price the standard Plus plan at $20 per month involved minimal testing. It seems the Pro plan’s pricing followed a similar approach.
Missteps like these are not unique to OpenAI. Pricing remains a complex challenge for many brands, especially in rapidly evolving industries like AI. But, with projected losses of $5 billion for 2024 and revenue of $3.7 billion, according to The New York Times, OpenAI’s case highlights the high stakes of getting it wrong. Effective pricing strategies require more than instinct – they rely on thoughtful market research and cost analysis to align with consumer expectations and business sustainability
The High Cost of Guesswork in Pricing
OpenAI has seen remarkable growth, with ChatGPT reaching 300 million weekly active users and earning its reputation as the gold standard in AI chatbots. Yet, this success is overshadowed by significant financial strain. Steep operational costs—driven by massive computational demands, data center investments, and energy consumption—have outpaced revenue, highlighting the perils of unsustainable pricing.
This situation underscores the importance of data-driven pricing strategies, especially for companies managing high-demand, high-cost products. OpenAI’s case shows that even the most innovative offerings can falter without a pricing model that accounts for operational realities and consumer behavior.
The company’s decision to adopt flat pricing reveals the risks of intuition-led strategies. While the $20 Plus plan and $200 Pro plan aimed to simplify access, they overlooked critical factors like regional affordability and usage intensity. As a result, the Pro subscription, tailored for power users, costs more to maintain than it generates in revenue—a problem amplified by the strain on computational resources.
Other tech giants have also struggled with pricing missteps. Take MoviePass, for example. The company famously offered unlimited movie tickets for $9.95 per month, far below the actual cost of a single ticket in most markets. The model led to a rapid influx of users but proved financially unsustainable, ultimately causing the company’s collapse. Similarly, Uber’s early ride-share pricing strategies ignored the long-term costs of driver incentives, leading to billions in losses as it fought to compete with rivals like Lyft.
Even in retail, companies have stumbled. JCPenney’s decision to eliminate sales and discounts in favor of “everyday low pricing” alienated loyal customers accustomed to frequent promotions. The misstep resulted in a significant revenue decline and a tarnished brand reputation.
For OpenAI, projected losses of $5 billion against $3.7 billion in revenue further emphasize the high stakes of getting pricing wrong. Without adjustments, ChatGPT’s unsustainable operational costs could undermine its long-term viability.
The lesson is clear: groundbreaking products, whether in AI, entertainment, or retail, can become financial liabilities without data-driven pricing strategies. Guesswork might deliver short-term gains but often leads to long-term instability. To thrive, businesses must align pricing with consumer behavior, regional realities, and operational costs—a task best accomplished through rigorous market research.
The Challenge of Fixed Global Pricing and Freemium Conversion
Fixed global pricing, such as the $20 ChatGPT Plus subscription, simplifies user acquisition but risks alienating users in lower-income regions where affordability varies. Tailored regional pricing could address these disparities, improving conversion rates and expanding the paying user base.
Additionally, OpenAI’s freemium model achieves a conversion rate of 5-6%, driving most of its revenue from subscriptions. However, sustaining growth in these figures demands deeper insights into user behavior. For example, which features encourage free users to convert? How do price thresholds differ for professional versus casual users? Robust market research could answer these questions, offering pathways to refine pricing and expand the paying user base.
How Market Research Could Have Informed OpenAI’s Pricing
OpenAI’s pricing challenges stem from a lack of market research. Methods like Gabor-Granger and Van Westendorp’s price sensitivity meter could have revealed the ‘sweet spot’ for balancing affordability and profitability.
By digging deeper into what users value, OpenAI could have tailored its tiers to appeal to different needs—without alienating heavy users or underserving casual ones. By leveraging these insights, OpenAI could have introduced pricing tiers that balanced accessibility and profitability across diverse user groups.
Market Research as the Key to Conversion
For OpenAI, converting free users to paid plans is both an opportunity and a challenge. With 5-6% of users upgrading, market research could uncover which features—affordability, advanced tools, or seamless access—drive these decisions. Techniques like conjoint analysis and A/B testing would provide valuable insights to align pricing and features with user needs, ensuring plans resonate with both casual and professional users.
Anticipating High Computational Costs
High operational costs, such as data center investments and energy consumption, drive OpenAI’s losses. Market research could have forecasted usage patterns to align pricing with demand, mitigating the strain of offering unlimited access to power users.
Testing Pricing Tiers Through Consumer Feedback
Testing pricing scenarios before launching the Plus and Pro tiers could have revealed acceptable price points, feature preferences, and perceived value through A/B testing and consumer feedback.
Bridging Global Markets and User Needs
Market research could have offered OpenAI critical insights to refine its global pricing strategy, aligning with regional purchasing power and user expectations. Techniques like Van Westendorp’s price sensitivity meter could have revealed pricing thresholds that resonate across diverse markets, striking a balance between accessibility and profitability.
Equally important is understanding the freemium user journey. Data-driven approaches like conjoint analysis would identify the features that drive free users to upgrade. Armed with these insights, OpenAI could have crafted subscription tiers that resonate with specific user segments, boosting conversion rates and ensuring sustainable revenue growth.
How Market Research Could Have Informed OpenAI’s Pricing
The challenges OpenAI faces with ChatGPT Pro’s pricing underscore the critical need for robust market research to guide financial decisions. By leveraging proven research methodologies, the company could have addressed key issues that now contribute to its financial strain.
Understanding User Segments and Price Sensitivity
Market research would have enabled OpenAI to segment its user base and assess each group’s willingness to pay for various subscription tiers. For instance:
- Casual Users: Individuals using ChatGPT for light, occasional tasks may prioritize affordability and would likely gravitate toward a lower-tier subscription.
- Power Users: Professionals, developers, or enterprises relying heavily on ChatGPT’s advanced features, like OpenAI o1 and Sora AI, may value efficiency and capabilities over price, making them more open to a higher-tiered model.
By understanding these distinctions, OpenAI could have introduced tailored pricing options that cater to specific needs while ensuring profitability.
Anticipating High Computational Costs
One of OpenAI’s greatest challenges is the high computational demand required to run ChatGPT. Market research could have helped forecast usage intensity across different user segments, providing critical data for pricing that aligns with operational costs. By factoring in expected usage patterns, OpenAI might have set higher prices or implemented limits for heavy users to balance the financial impact of intensive computational loads.
Testing Pricing Tiers Through Consumer Feedback
Before launching its Plus and Pro subscription models, OpenAI could have employed targeted market research to test pricing tiers and identify optimal price points. Techniques such as A/B testing would have allowed the company to evaluate real-world reactions to various pricing combinations, ensuring that the final structure resonated with users while covering costs.
Proven Market Research Techniques
- Gabor-Granger Technique: OpenAI could have directly assessed users’ willingness to pay for features included in ChatGPT Pro. Respondents would be presented with different price points, and their responses would help identify the price elasticity of demand, highlighting a sustainable price range for the subscription.
- Van Westendorp Price Sensitivity Meter: This technique could have gauged customer perceptions of pricing ranges, determining the “too cheap,” “too expensive,” and “just right” price thresholds. OpenAI could have used this data to position its Pro offering at a price seen as both premium and fair, avoiding alienation while maximizing revenue potential.
Rethinking the Pricing Model
Sam Altman’s recent suggestion of a potential shift to usage-based pricing reflects an acknowledgment that the current flat-rate subscription model may not be sustainable. Transitioning to a usage-based or hybrid pricing model could offer a path to profitability, but success depends on understanding user behavior and pricing thresholds – a task ideally suited for market research.
Identifying Willingness to Pay for Additional Features
Market research can help pinpoint where users find value in additional features or increased computational power, guiding the creation of scalable pricing. For instance:
- Power users, such as businesses or developers, may be willing to pay more for advanced capabilities like OpenAI o1 or Sora AI video generation.
- Casual users might prioritize affordability but could accept additional costs for occasional access to premium features.
Techniques like conjoint analysis could evaluate trade-offs users are willing to make, helping OpenAI determine the features that justify higher pricing.
Balancing Accessibility with Profitability
Usage-based pricing introduces a challenge: ensuring accessibility for casual users while maintaining profitability from heavy users. Market research could map out demand curves, revealing usage patterns and helping establish fair thresholds. For example:
- Light users might benefit from a pay-as-you-go model, ensuring they only pay for what they use.
- Heavy users, who consume significant computational resources, could be charged progressively higher rates as usage increases, aligning costs with revenue.
Through techniques like surveys and simulations, OpenAI could test user responses to proposed pricing structures, minimizing backlash while maintaining equitable access.
Exploring Tiered Pricing Models
Tiered pricing, informed by market research, could provide flexibility for different user segments without alienating any group. For example:
- A Basic Tier for casual users, offering limited access at a low price.
- A Pro Tier for professionals and power users, with expanded features and higher computational allowances.
- An Enterprise Tier for organizations, offering custom solutions based on usage and specific needs.
Each tier could be tested through pilot programs or focus groups to assess demand and fine-tune features and pricing. Techniques like Gabor-Granger or Van Westendorp could ensure each tier aligns with user expectations and willingness to pay.
From Flat Rates to Tailored Solutions
By integrating market research into its pricing strategy, OpenAI could shift from a one-size-fits-all model to a flexible system that reflects user needs and operational realities. Whether adopting usage-based, tiered, or hybrid pricing, the goal remains the same: aligning value with cost to create a sustainable and scalable model that works for both users and the company.
Final Thoughts: Lessons for CEOs and Brands
OpenAI’s pricing missteps provide a powerful case study on the critical importance of data-driven decision-making in today’s complex and competitive markets. Despite its innovations and rapid user growth, OpenAI’s reliance on intuition over data has caused financial strain. The lesson for leaders across industries is clear: structured analysis is essential.
First, pricing is not just about setting numbers—it is a strategic lever that impacts profitability, accessibility, and user satisfaction. Companies must move beyond assumptions or limited testing and instead leverage robust market research to understand consumer behavior, willingness to pay, and regional dynamics. Techniques such as the Gabor-Granger and Van Westendorp methods offer precise data on pricing thresholds, while conjoint analysis and A/B testing can uncover which features users value most.
Second, market research is not a one-time activity. Regularly revisiting pricing strategies is essential to stay aligned with evolving consumer preferences, market conditions, and operational realities. As OpenAI’s case demonstrates, even the most innovative offerings can become unsustainable if they fail to account for high operational costs or diverse user needs. Tools like usage-based or tiered pricing models, informed by ongoing research, can create equitable solutions for both light and heavy users.
Third, the freemium model is both an opportunity and a challenge. OpenAI’s 5-6% conversion rate is a testament to the potential of free-to-paid upgrades, but sustaining and growing these figures requires deeper insights into user behavior. Understanding what drives conversions—whether it’s affordability, premium features, or seamless access—is key to designing subscription tiers that resonate with different segments.
Finally, visionary leadership is strengthened by structured decision-making. While intuition and bold moves often define industry leaders, the best outcomes are achieved when those instincts are paired with disciplined analysis. Investing in the right tools, teams, and methodologies for market research ensures that every decision is grounded in actionable insights.
OpenAI’s experience underscores that pricing is not merely a financial consideration—it’s a strategic cornerstone of long-term success. For business leaders navigating similar challenges, the takeaway is clear: in an increasingly complex market, thriving requires more than innovation; it demands a commitment to data-driven strategies that align user expectations with business realities.
Get regular insights
Keep up to date with the latest insights from our research as well as all our company news in our free monthly newsletter.
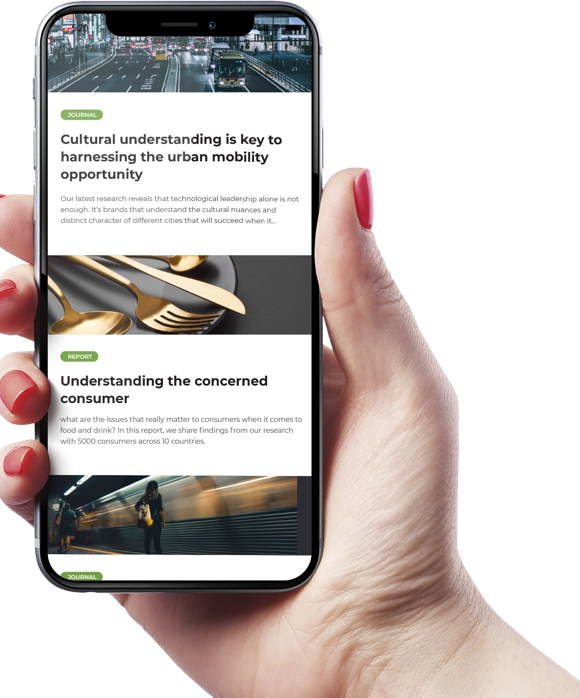