What would happen if market researchers borrowed a lens from data science?
The traditional tools of market research are fast becoming quaint relics. The old methods of garnering consumer insights and market trends are increasingly seen as mere snapshots that lack the dynamic and predictive capabilities essential in today’s business environment.
This evolving scenario beckons a new way for market researchers to transcend traditional boundaries and imbibe the analytical and predictive prowess inherent in data science.
The argument is simple yet profound: for market research to remain a potent tool for organizational success, it must evolve by adopting a data science mindset.
Data science’s methodology is indispensable for effective market research. The exigencies of predictive analysis, the power of machine learning, and the profound impact of a data-informed market strategy are reshaping brands.
The symbiotic relationship between market research and data science is bound to unfold as a critical imperative for market researchers worldwide.
Transitioning Beyond Traditional Market Research
Traditionally, market research was built mainly on surveys, focus groups, and observational methods, although solid, now demands more sophisticated analytical layers to unravel market complexities.
The archaic method of merely ‘asking’ consumers has shown its limitations in a world where consumers’ preferences change at the drop of a hat. The depth and breadth of insights required to navigate modern market intricacies necessitate a more robust, analytical, and predictive approach – hallmarks of data science.
The Changing Landscape of Market Research
Traditional market research has its roots in qualitative methodologies—focus groups, in-depth interviews, and surveys. These methods have been crucial in gathering deep insights into human behaviors and motivations.
However, the digital age presents a deluge of data, with machine learning, advanced algorithms, and artificial intelligence leading the charge. This shift means market researchers must adapt, incorporating these advanced techniques, not as a replacement but as an extension of their existing methodologies.
The fusion of traditional research with digital methodologies offers a comprehensive understanding. As a result, market researchers are now positioned at a crucial crossroads where merging past practices with present technological advancements is essential for future success.
As we forge into a world of data overload and advanced analytics, market research stands at the cusp of a profound metamorphosis. This transformation is instigated by the amalgamation of robust technological advancements, including Big Data, machine learning, and artificial intelligence.
These technological enablers are redefining the perimeter of what’s possible within market research, extending it beyond its traditional contours.
The Advent of Big Data
The entry of Big Data into the market research field has redefined the scale and scope of available market intelligence. Unlike in the past, where data was often limited and collected through traditional methods, today’s market researchers have access to an almost inexhaustible reservoir of data.
This data captures a snapshot of consumer behavior and forms a continuous stream of insights, providing a dynamic and real-time understanding of market trends. The vast amount of data can be analyzed to unearth hidden patterns, consumer sentiments, and emerging market opportunities, thereby providing a richer, more nuanced understanding of the market.
The Machine Learning (ML) Leverage
Machine Learning (ML), a subset of artificial intelligence, is acting as a catalyst in evolving the methods of market research.
Where traditional methods often rely on static data and provide a retrospective view of market trends, ML employs algorithms that learn and improve with data, offering predictive insights and real-time analysis.
For instance, ML-powered predictive analytics can help market researchers forecast market trends, consumer behavior, and the impact of various factors on market dynamics. The integration of machine learning transforms market research from a reactive to a proactive function, enabling businesses to stay ahead of the curve.
The Altered Role of Market Researchers
With these technological advancements, market researchers’ role is evolving from mere data gatherers to insightful analysts and strategic advisors.
The quintessence of market research now lies in harnessing the power of advanced analytics to translate data into actionable insights. This transition mandates a higher level of technical acumen, a grasp of analytical tools, and an understanding of data management.
The function of market researchers now intertwines with data science, requiring a blend of skills to navigate the complex data ecosystem.
The Shift in Methodology:
The methodology in market research is also witnessing a tectonic shift. Traditional methods like surveys and focus groups are now being augmented or even replaced by data-driven approaches.
Techniques such as sentiment analysis, predictive modeling, and data mining are becoming commonplace, marking a significant leap in the analytical rigor of market research. These methods allow for a more comprehensive and nuanced understanding of markets, enabling better decision-making and strategy formulation.
Market research is expanding, propelled by the winds of technological change and advancements. As these changes crystallize, the infusion of a data science mindset in market researchers is desirable and indispensable for navigating the complex market landscape.
The new stage of market research beckons a breed of market researchers—ones who are not only adept in traditional research methods but are also proficient in leveraging the burgeoning power of data science to drive market understanding to new frontiers.
Why Market Researchers Should Think Like Data Scientists
The imperative for market researchers to morph their thought processes and methodologies along the lines of data science stems from the inexorable shift toward a data-driven business ecosystem.
Market researchers now find themselves at the crossroads where the old must be melded with the new to extract richer, deeper insights from a sea of data that washes over the market.
This transformation is supported by the infusion of specific quintessential characteristics intrinsic to data scientists, which are now recognized as invaluable for modern market research.
Here’s an exploration of these critical traits:
Curiosity
At the heart of a data scientist’s disposition lies an insatiable curiosity—a relentless quest to unearth the ‘why’ and ‘how’ behind data. This goes beyond the surface-level insights, delving into the underlying patterns, behaviors, and trends.
Market researchers with a similar level of curiosity can decode complex market dynamics, which empowers organizations to understand better and cater to their target audiences. By inculcating a data scientist’s curiosity, market researchers can transform raw data into a goldmine of insights, leading to more informed and strategic business decisions.
Statistical Knowledge
A robust understanding of statistical models is the bedrock of effective data analysis. Data scientists leverage statistical knowledge to validate findings, ensure accuracy, and derive insights from data. For market researchers, honing statistical skills enables a more rigorous analysis of market data, making insights more reliable and actionable.
Through a firm grasp of statistical models, market researchers can delve into data, recognize and validate trends, and provide a solid foundation for market strategies.
Programming Skills
In a data-laden world, automating processes and manipulating data through coding is a significant asset. Programming skills enable data scientists to manage large datasets efficiently, extract relevant information, and automate repetitive tasks.
By acquiring programming skills, market researchers can significantly enhance their ability to handle large datasets and automate data collection and analysis processes, making market research operations more efficient and insightful.
This also opens up new avenues for complex analysis that can be invaluable in understanding intricate market dynamics.
Business Acumen
Transcending the technical sphere, a data scientist’s ability to translate complex data insights into actionable business strategies is a cornerstone of their organizational value. Similarly, market researchers must evolve from mere data collectors to strategic advisors.
With enhanced business acumen, market researchers can interpret data in the context of broader business goals and market strategies. This ability to translate data into actionable business insights elevates market research from a supportive function to a strategic one.
The Intersection of Market Research and Data Science
The modern marketplace is an evolving canvas of consumer behaviors, preferences, and global trends. Navigating this dynamic age necessitates a fusion of market research and data science, heralding a new era where potent data science techniques augment traditional market research methodologies.
The potential to extract deeper, more nuanced insights through data science techniques propels market research into new possibilities. As brands grapple with an ever-complex market, the fusion of market research and data science emerges as a beacon of insight, strategy, and competitive advantage.
Through this symbiotic relationship, market research is not just keeping pace with the evolving market dynamics. Still, it is poised to stay ahead, making it an invaluable asset in the organizational arsenal.
As these two fields converge, several cutting-edge techniques emerge.
This intersection is forging innovative pathways to explore market dynamics with a depth and precision erstwhile unimagined. Here are some of the characteristic data science techniques and their applications in market research:
Machine Learning for Customer Segmentation
Machine Learning (ML), a subset of artificial intelligence, is a powerhouse of analytics that thrives on data to generate insights.
One of the stellar applications of ML in market research is customer segmentation. By leveraging unsupervised learning algorithms, market researchers can dissect a heterogeneous market into homogeneous customer segments.
Each segment, characterized by similar behaviors or preferences, provides a nuanced understanding of diverse customer personas.
This granularity in segmentation facilitates personalized marketing strategies, optimizing resource allocation, and enhancing customer engagement, which is indispensable for achieving a competitive edge in today’s hyper-competitive markets.
Natural Language Processing for Social Media Analysis
The rise of social media has bestowed market researchers with a treasure trove of unstructured data that encapsulates consumer sentiments, opinions, and trends.
Natural Language Processing (NLP), a discipline within artificial intelligence, specializes in deriving actionable insights from textual data. Through sentiment analysis, topic modeling, and other NLP techniques, market researchers can delve into social media chatter to gauge public sentiment, identify emerging trends, and detect potential crises.
This real-time social media analysis via NLP transforms market research into a dynamic tool that keeps the pulse on market sentiments, empowering brands with actionable insights to tailor their strategies accordingly.
Predictive Analysis for Future Trends
Predictive analysis is a lighthouse in turbulent seas, providing foresight that can be leveraged for strategic advantage. Using statistical and machine learning algorithms, predictive analysis can forecast future market trends, consumer behaviors, and potential market disruptions.
For market researchers, this goes beyond the traditional reactive analysis, propelling market research into a proactive strategic function. Predictive models, built on historical and real-time data, provide a foresight indispensable for strategic planning, risk mitigation, and seizing market opportunities before competitors.
How to Adopt a Data Science Mindset
Infusing data science into market research is more than a mere marriage of methodologies—it’s a mindset. Transitioning into this enriched analytical strategy necessitates a blend of education, practical exposure, and a dive into tools that are staples in data science.
Here’s a structured pathway for market researchers to imbibe a data science mindset:
Educational Foundation:
- Formal Education: Pursuing courses or certifications in data science, statistics, machine learning, and other related fields. Renowned platforms like Coursera, edX, or university programs offer comprehensive courses that provide a solid foundation in data science principles.
- Online Resources: There is a wealth of online resources, including MOOCs (Massive Open Online Courses), webinars, and tutorials available for self-learners. Websites like DataCamp and Kaggle offer interactive lessons and challenges that provide practical exposure to data science concepts.
Hands-On Experience:
- Project Involvement: Engaging in internal projects where data science techniques are employed is a great way to gain practical experience. Collaborating with data science teams and working on real-world problems will enhance understanding and application.
- Competitions and Hackathons: Participating in data science competitions or hackathons provides a platform to apply learned skills, interact with other data science enthusiasts, and gain feedback on your approach and solutions.
Tool Proficiency:
- Python: A versatile, widely-used programming language in the data science community. Learning Python is fundamental as it is employed for various data analysis, machine learning, and statistical tasks.
- R: Another powerful language and environment for statistical computing and graphics. R is extensively used for statistical analysis and visualizing data.
- SQL: Acquiring skills in SQL is crucial for data manipulation and retrieval from relational database management systems.
Networking and Community Engagement:
- Meetups and Conferences: Attending data science meetup conferences and engaging with the community provides exposure to the latest trends, tools, and best practices in data science.
- Online Forums: Engaging in forums like Stack Overflow or the Data Science Stack Exchange can accelerate learning through interaction with seasoned professionals.
Continuous Learning and Adaptation:
- Keep Abreast of Emerging Trends: Data Science is a rapidly evolving field. Staying updated on the latest algorithms, tools, and industry applications is essential.
- Feedback and Iteration: Seeking feedback from data science professionals and continuously improving through iterative learning and practice is key to mastery.
Collaborative Learning:
- Study Groups: Forming or joining study groups with peers interested in data science can foster a collaborative learning environment.
- Mentorship: Seeking mentorship from seasoned data science professionals can provide guidance and feedback and accelerate the learning curve.
Benefits and Limitations of Merging Market Research with Data Science Principles
Harnessing data science in market research undoubtedly offers enriched insights and proactive strategies. However, it’s essential to navigate with caution.
As we increasingly rely on data, issues like data privacy, security breaches, and ethical considerations become paramount. While data can reveal much, the emotional, intangible aspects of consumer behavior might still be best understood through traditional methods.
The fusion of market research and data science heralds a new era of enriched insights and strategic foresight. A nuanced understanding of both can prepare organizations to navigate this integration more effectively.
The Benefits:
Deeper Insights:
- Merging data science with market research allows for an in-depth analysis of market dynamics, consumer behaviors, and emerging trends. The quantitative rigor of data science complements the qualitative insightfulness of market research, creating a richer analytical lens.
Predictive Capabilities:
- Data science methodologies like predictive analytics empower market researchers with the ability to forecast market trends and consumer behaviors with a higher degree of accuracy, which is instrumental in proactive strategy formulation.
Efficiency and Automation:
- Automation of data collection, processing, and basic analysis through programming and machine learning algorithms significantly enhances the efficiency of market research operations, allowing for quicker turnarounds and timely insights.
Enhanced Decision-Making:
- Combining traditional market research expertise and data science’s quantitative analysis results in a robust decision-making framework, enabling organizations to make well-informed, data-driven decisions.
Customization and Personalization:
- Data science facilitates a granular understanding of customer segments, which is pivotal for tailored marketing strategies and personalized customer experiences, driving enhanced engagement and loyalty.
Competitive Advantage:
- Organizations employing a data science approach in market research are better positioned to glean actionable insights that can provide a competitive advantage in the rapidly evolving market landscape.
Limitations:
Data Privacy Concerns:
- The extensive data collection and analysis inherent in data science applications raise serious privacy concerns. Ensuring compliance with burgeoning data privacy regulations across different regions is a significant challenge.
Risk of Over-Complication:
- There’s a potential risk of over-complicating simple issues through excessive reliance on complex data science models, which might lead to paralysis by analysis or misguided strategies.
Skill Gap:
- The transition requires a substantial upskilling of the market research workforce in data science principles, which demands time, resources, and a long-term commitment.
Quality of Data:
- The accuracy and reliability of insights derived through data science are heavily contingent on the quality and relevance of data. Inadequate or incorrect data can lead to misguided insights and decisions.
Technology Investment:
- Significant investment in technology infrastructure and tools is necessary to facilitate the integration of data science in market research, which might pose a financial challenge for some organizations.
Resistance to Change:
- Traditional market researchers might resist the new data-driven approach, necessitating a cultural shift and a change management strategy to ensure smooth integration.
Final Thoughts
The profound impact of the integration of market research and data science has a profound impact on organizational decision-making, competitive positioning, and strategic foresight.
However, like any ambitious endeavor, this integration does not have challenges. Data privacy concerns, the risk of over-complication, and the requisite investment in skills and technology present hurdles that necessitate careful consideration and strategic planning.
The compelling advantages, however, far outweigh the challenges, and market researchers must seize this opportunity to upskill, broaden their analytical horizons, and embrace the data science mindset to drive deeper insights and innovation.
Get regular insights
Keep up to date with the latest insights from our research as well as all our company news in our free monthly newsletter.
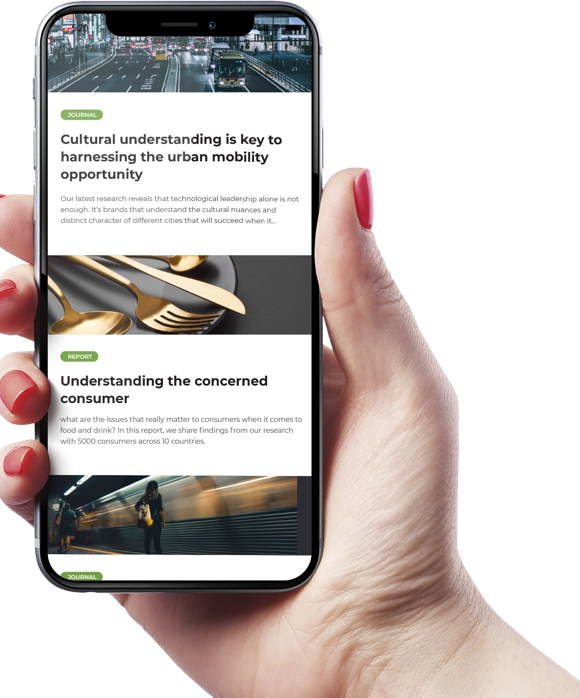