In today’s world, data has become an essential asset for businesses. However, collecting data alone is insufficient; it must be analyzed and turned into meaningful insights. This is where predictive analytics comes in.
Predictive analytics is the use of statistical algorithms, machine learning, and data mining techniques to analyze historical data and make predictions about future events or trends.
Predictive analytics has been around for a long time, with roots dating back to the early 1800s. One of the earliest known examples of predictive analytics is the work of the English statistician Francis Galton, who used statistical techniques to predict the height of children based on the height of their parents. Since then, predictive analytics has evolved significantly and is now a critical component of modern business intelligence.
Predictive analytics has many names, such as advanced analytics, data mining, and machine learning. However, they all refer to the same basic concept of using data to make predictions.
The importance of predictive analytics in market research cannot be overstated. With the abundance of data available today, businesses need to be able to make informed decisions quickly to stay ahead of the competition. Predictive analytics can help companies to identify trends, predict customer behavior, and optimize pricing strategies. According to a survey by McKinsey & Company, companies that use predictive analytics are twice as likely to be in the top quartile of financial performance within their industry.
Get regular insights
Keep up to date with the latest insights from our research as well as all our company news in our free monthly newsletter.
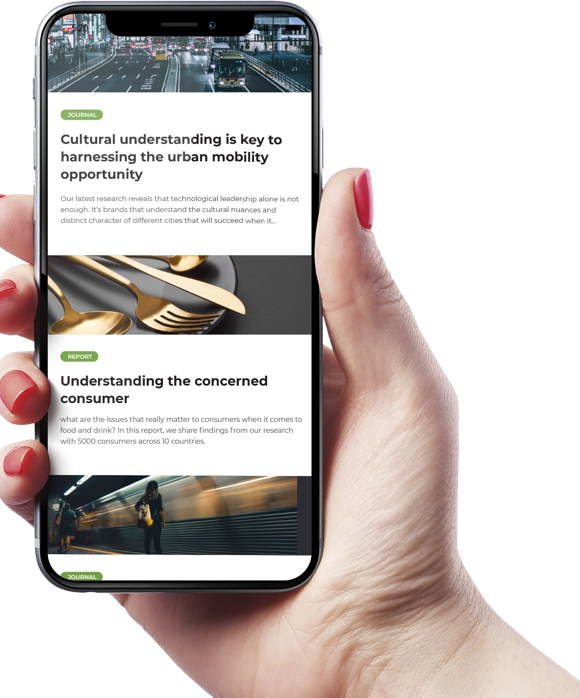
The Importance of Predictive Analytics in Market Research
The importance of predictive analytics in market research lies in its ability to provide businesses with the insights they need to make informed decisions and stay ahead of the competition. Brands can predict future behavior and adjust their strategies by analyzing historical data and identifying patterns and trends.
One example of the power of predictive analytics is the case of Target, a large retail chain. Target analyzed its customers’ purchasing patterns and used that data to predict when customers were most likely to become pregnant. With this information, Target could send targeted advertisements and coupons to these customers, increasing sales and customer loyalty.
Another real-world example is how predictive analytics helped the Seattle Seahawks win the Super Bowl in 2014. The team used predictive analytics to analyze their opponents’ behavior and tendencies, allowing them to make strategic decisions during the game.
According to a study by Forbes Insights, businesses that use predictive analytics are more likely to experience improved customer engagement, increased profitability, and better overall business performance.
Benefits of Predictive Analytics in Market Research
The benefits of predictive analytics in market research are numerous, and businesses that use this technique can gain valuable insights that can inform their decision-making process. According to a study by Harvard Business Review, businesses that use predictive analytics are more likely to experience increased revenue and profitability.
Here are some of the key benefits of using predictive analytics in market research:
- Improved accuracy in forecasting: Predictive analytics can help businesses forecast future trends and outcomes with a high degree of accuracy. This can help brands better plan their operations and resources to meet future demands. For example, a hotel chain can use predictive analytics to forecast room occupancy rates, allowing them to adjust staffing and inventory levels accordingly.
- Identifying trends: Predictive analytics can help businesses identify trends in customer behavior, market conditions, and more. By identifying these trends, companies can adapt their strategies to meet changing market conditions. For example, a retail business can use predictive analytics to identify emerging customer purchasing behavior trends, allowing it to adjust its inventory accordingly.
- Predicting customer behavior: Predictive analytics can help businesses predict customer behavior, such as buying patterns, preferences, and responses to marketing campaigns. This can help companies to tailor their marketing efforts and improve customer engagement. For example, an e-commerce business can use predictive analytics to identify customers who are most likely to make a purchase, allowing them to target these customers with personalized offers.
- Optimizing pricing strategies: Predictive analytics can help businesses optimize their pricing strategies by identifying the optimal price point for products and services. Using predictive analytics, brands can adjust their pricing strategies to maximize profits and stay competitive. For example, an airline can use predictive analytics to adjust ticket prices based on demand, maximizing revenue while ensuring seats are filled.
Use Cases of Predictive Analytics Around the World
Brands across the globe are increasingly using predictive analytics to gain insights into market trends and customer behavior. Here are some examples of how businesses have used predictive analytics:
- Tesco – a leading UK-based grocery retailer, used predictive analytics to identify the most profitable products and services for their customers. By analyzing customer data, Tesco was able to tailor its offerings to meet the specific needs of its customers, resulting in increased sales and customer loyalty.
- Amazon – the world’s largest online retailer, uses predictive analytics to provide personalized recommendations to customers. By analyzing customer data, Amazon can recommend products and services most relevant to each customer, increasing sales and customer satisfaction.
- Alibaba – one of China’s largest e-commerce companies, uses predictive analytics to identify products likely to be popular with customers. By analyzing search and purchase data, Alibaba can recommend products and services that are most likely to become successful, leading to increased sales and revenue.
- Toyota – a leading automobile manufacturer, uses predictive analytics to identify the most profitable sales channels and to optimize pricing strategies. Toyota can adjust its pricing strategies by analyzing sales data to maximize profits and stay competitive.
- Tokopedia – a leading e-commerce platform in Indonesia, uses predictive analytics to identify popular products and optimize pricing strategies. By analyzing customer data, Tokopedia can adjust its pricing strategies to meet customer demand, leading to increased sales and revenue.
These examples show how businesses in various countries leverage the power of predictive analytics in market research to achieve their goals, such as increasing sales, improving customer satisfaction, and staying ahead of the competition.
Challenges of Predictive Analytics
While predictive analytics can be a powerful tool for brands, it’s essential to understand the challenges associated with using this technique.
According to a study by McKinsey & Company, many businesses struggle with these challenges when implementing predictive analytics. For example, the study found that only 19% of companies are very confident in the accuracy of their predictive models.
Here are some of the challenges of using predictive analytics in market research:
- The need for large amounts of data: To accurately predict future outcomes and trends, businesses need large quantities of high-quality data. This can be a challenge for companies that don’t have access to the necessary data or that struggle with data quality issues.
- Potential for biases in data analysis: Predictive analytics is only as good as the data used to train the models. If the data used to train the model is biased, the predictions made by the model will also be biased. For example, a predictive model that is trained using only data from a specific demographic may not accurately predict behavior for other demographics.
- Difficulty in interpreting results: Predictive analytics can provide businesses with a large amount of data and insights, but it can be challenging to interpret these results and turn them into actionable strategies. Companies need the necessary skills and expertise to interpret the data and make informed decisions.
- Data privacy and security concerns: As businesses collect more data for predictive analytics, there are concerns about data privacy and security. Companies must comply with data protection regulations and take appropriate measures to secure their data.
As Dr. Michael Wu, Chief AI Strategist at PROS, said, “The biggest challenge in predictive analytics is not the algorithm, but the data.” To overcome the challenges of using predictive analytics in market research, businesses must invest in data quality and security and ensure they have the necessary skills and expertise to interpret the data and make informed decisions.
Best Practices for Implementing Predictive Analytics
To successfully implement predictive analytics, businesses must follow best practices to ensure they get the most out of this powerful tool. Here are some tips and best practices for companies looking to implement predictive analytics in their market research:
- Choose the right software tools: Many software tools are available for predictive analytics, and businesses must choose the one that best meets their needs. This can include tools that provide data visualization, machine learning algorithms, and data cleaning and preprocessing.
- Ensure data quality: As discussed earlier, data quality is critical for accurate predictions. Businesses must ensure they have high-quality data, free from errors and biases. This can include data cleaning, normalization, and validation.
- Involve domain experts: Domain experts, such as market research analysts, can provide valuable insights and context for the data used in predictive analytics. By involving these experts in the process, businesses can ensure that their predictions are relevant and actionable.
- Use historical data: Predictive analytics relies on historical data to make predictions about the future. Businesses need to have access to historical data, which should be relevant to the problem being addressed.
- Test and refine the model: Predictive models should be tested and refined to ensure accuracy and reliability. This can involve using different algorithms, adjusting parameters, and comparing the results to actual outcomes.
- Monitor and update the model: Predictive models should be monitored and updated over time to remain relevant and accurate. As market conditions change, the model may need to be updated to reflect these changes.
According to a study by the International Institute for Analytics, businesses that follow best practices for implementing predictive analytics are more likely to succeed. For example, the study found that brands involving domain experts in the process are more likely to see positive results.
By following these best practices, businesses can ensure they make the most of predictive analytics in their market research efforts.
In conclusion, predictive analytics is a powerful tool for businesses seeking insights into market trends and customer behavior. Companies can use historical data and machine learning algorithms to predict future outcomes and adjust their strategies accordingly. However, there are challenges associated with using predictive analytics, such as the need for large amounts of high-quality data and the potential for biases in data analysis.
To successfully implement predictive analytics in market research, businesses must follow best practices, such as choosing the right software tools and involving domain experts.
Kadence International, a market research agency, can help businesses navigate market research challenges and leverage the power of predictive analytics. With data collection, analysis, and interpretation expertise, we can provide valuable insights and help brands make data-driven decisions that lead to success. Contact us today to learn how we can help your business with market research.