Did you know that, on average, shoppers spend just two seconds deciding whether to pick up a product or not? In that short amount of time, packaging has to grab their attention, communicate key information, and entice them to purchase. As a marketer, understanding the psychology behind the packaging is essential for capturing those fleeting moments and making the most of your opportunity on the shelf. In this blog, we’ll explore the fascinating research into shopping behaviour and eye-tracking studies and show you how to design packaging that influences consumers’ decisions. So, if you want to know the secrets to gain consumers’ attention from a shelf, keep reading!
The Science of Shopping Behavior
To create effective packaging, it’s essential to understand how shoppers behave in a store. Numerous studies into shopping behaviour offer key insights into how to design packaging that resonates with your target audience.
One important insight is that shoppers tend to make decisions based on emotion rather than logic. Packaging that conveys a sense of excitement, pleasure, or indulgence is more likely to attract their attention than packaging that simply lists the product’s features.
Another crucial insight is that shoppers look at a product’s upper left corner first. This area should contain the most vital information, such as the product’s name or a key benefit.
It is important to note that this insight is based on eye-tracking studies conducted primarily in Western societies, such as the United States and Europe. Shoppers in other countries may have different eye-tracking patterns or prioritise different areas of a product’s packaging.
Finally, shoppers prefer products that are easy to understand and use. Clear and concise communication on packaging regarding the product and its usage will help the product stand out on the shelf.
Get regular insights
Keep up to date with the latest insights from our research as well as all our company news in our free monthly newsletter.
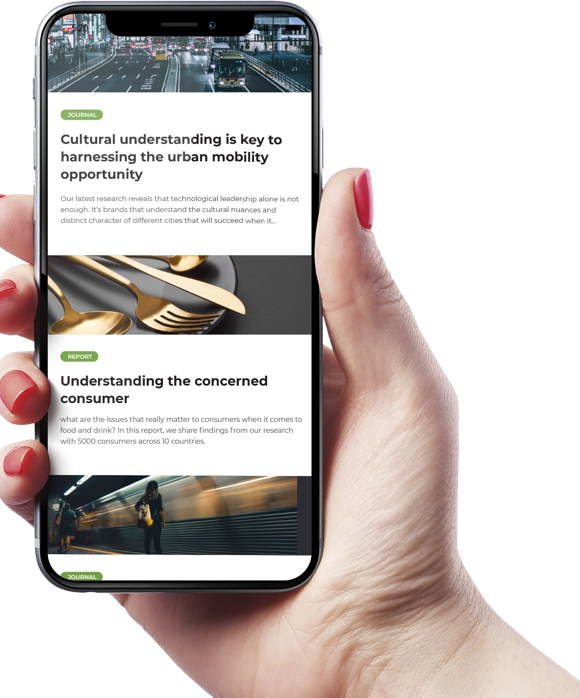
The Power of Eye-Tracking Studies
While studies into shopping behaviour can provide valuable insights, they can also be limited by the self-reported nature of the data. Researchers have turned to eye-tracking studies to get a more accurate picture of how shoppers behave in-store.
Eye-tracking technology allows researchers to see where shoppers are looking and for how long. This provides a more objective way of measuring shopper behaviour and can reveal insights that might not be captured through self-reported data.
One key finding from eye-tracking studies is that shoppers focus on the front of the package first, then move on to the sides and back. That means that the front of your package needs to be eye-catching and convey essential information clearly and concisely.
Another important insight from eye-tracking studies is that shoppers tend to look at products at eye level more than those that are higher or lower. If your product is on a lower or higher shelf, you may need to use packaging design elements that stand out even more to attract attention.
Eye-tracking studies can also reveal how shoppers scan a package for information. For example, they tend to look at the product name, the image or graphic, and then any claims or benefits listed on the front of the package.
By using the insights from eye-tracking studies, you can design packaging that is even more effective at attracting attention and communicating key information to your target audience.
Designing Packaging to Stand Out
Now that we better understand how shoppers behave in-store and the insights gained from eye-tracking studies, let’s explore some specific design elements that can help your packaging stand out on the shelf.
Colour
Colour is one of the most powerful design elements for attracting attention. Using bold and bright colours can help your product stand out. Consider using colours not commonly seen in your product category to make your product even more distinctive.
However, colour can be perceived differently in different countries, and marketers need to be aware of these differences when designing packaging for a global audience. For example, in Western cultures, black is often associated with luxury and sophistication, while in some Eastern cultures, it is associated with mourning and sadness. Similarly, the colour red is often associated with love and passion in Western cultures, while in some Asian cultures, it is associated with luck and prosperity.
It’s also important to consider the context in which the product will be sold, as colours can have different meanings in different contexts. For example, green may be associated with nature and health in some contexts, but it may relate to money and finance in others. By carefully considering the cultural and contextual implications of colour, marketers can create packaging that effectively communicates the product’s value and resonates with the target audience.
Typography
Typography refers to the style, size, and arrangement of text on your packaging. Using clear and easy-to-read fonts can help shoppers quickly understand what your product is and what it offers. It is also important to note there can be differences in buyer behaviour and preferences regarding typography in different cultures.
For example, in many Asian cultures, calligraphy and other forms of intricate handwriting are highly valued, and this may influence the types of typography that are preferred by consumers. Similarly, different scripts and writing systems may have different connotations and associations in different cultures, impacting buyer behaviour.
It’s worth noting that typography can also significantly impact accessibility and readability for people with visual impairments or other disabilities. Designing clear and easy-to-read typography can help ensure your product is accessible to the broadest possible audience, regardless of cultural or linguistic background.
Imagery
Compelling imagery can help your product stand out and connect with shoppers emotionally. Consider using high-quality photos or illustrations that convey the benefit or feeling your product provides.
Packaging Shape
The shape of your packaging can also help it stand out on the shelf. Consider using unique shapes or structures different from the typical packaging in your product category.
Branding
Finally, branding can also play a crucial role in attracting attention and building loyalty. Ensure your packaging design is consistent with your brand identity and conveys your brand values.
Real-World Examples of Successful Packaging Design
One of the best ways to learn about effective packaging design is to look at real-world examples. Here are a few successful packaging designs that have used the principles we’ve discussed:
- Burt’s Bees: Burt’s Bees packaging stands out on the shelf with its natural, earthy colours and simple, elegant typography. Using natural images and illustrations of bees and flowers helps to communicate the brand’s commitment to using natural ingredients.
- Oatly: Oatly’s packaging for their dairy-free milk products stands out on the shelf with its bold use of typography and graphics. The packaging features a simple black and white design with clever and irreverent messaging, such as “It’s like milk, but made for humans.”
- Chobani: Chobani’s yoghurt packaging features a distinctive, curved shape that differentiates it from other yoghurt brands. The packaging also features bold, colourful graphics and typography that help it stand out on the shelf.
- Method: Method’s cleaning product packaging features bright, cheerful colours and playful illustrations that help it stand out from the typically bland and boring cleaning products. The packaging also features witty product names that add to the brand’s playful personality.
- Nivea: Nivea’s skincare packaging features a simple, classic design that has become synonymous with the brand. The packaging features a clean, white background with the brand’s iconic blue logo, which helps it stand out on the shelf and communicate its commitment to quality skincare products.
A Case Study on Packaging that Missed its Mark
While the above are great examples of packaging that resonate well with buyers, marketers can also learn from many packaging failures.
Case Study: Bic For Her
In 2012, Bic introduced a line of pens called “Bic For Her,” marketed as pens designed specifically for women. The pens featured pastel colours and a thinner barrel size and were priced higher than regular pens. The packaging also included a tagline “Designed to fit comfortably in a woman’s hand.”
The product was met with widespread criticism and mockery on social media, with many people questioning why women would need pens explicitly designed for them. Some critics also pointed out that the pens were more expensive than regular pens, despite offering no significant additional features or benefits.
There are several steps that Bic could have taken to avoid the Bic For Her disaster. Here are a few possible strategies:
- Conduct Research: Before launching a new product, it’s essential to conduct thorough market research to understand the needs and preferences of your target audience. In the case of Bic For Her, Bic could have conducted surveys or focus groups to better understand whether there was a demand for pens designed specifically for women.
- Avoid Stereotypes: The marketing of Bic For Her relied heavily on gender stereotypes, such as the idea that women have delicate hands that require special pens. To avoid this, Bic could have focused on creating marketing messages that were more inclusive and resonated with a diverse range of consumers.
- Price the Product Appropriately: One of the criticisms of Bic For Her was that the pens were priced higher than regular pens, despite offering no significant additional features or benefits. To avoid this, Bic could have priced the product more competitively or provided clear and compelling reasons why the pens were worth the higher price.
- Test the Product: Before launching a new product, testing it with a smaller audience is vital to see how it is received. In the case of Bic For Her, Bic could have tested the pens with a smaller group of consumers to see whether the product resonated with them before launching it on a larger scale.
- Learn from Feedback: When the negative feedback about Bic For Her started to emerge, Bic could have responded more quickly and effectively to address the concerns.
By taking these steps, Bic could have avoided the Bic For Her disaster and created a product that resonated with consumers and drove sales. The key is to understand your target audience, create marketing messages that are inclusive and relevant, and be responsive to feedback and criticism when it arises.
While Bic For Her was widely criticised, the brand was able to learn from its mistakes and move forward. In subsequent marketing campaigns, Bic focused on creating messages that resonated with all consumers, regardless of gender. By acknowledging their missteps and making changes based on feedback, Bic was able to salvage its brand reputation and avoid making similar mistakes in the future.
Putting It All Together
Now that we’ve explored the psychology of shopping behaviour, the power of eye-tracking studies, and specific design elements that make packaging stand out, let’s bring it all together.
A deep understanding of your target audience is essential to create effective packaging. What are their values, preferences, and pain points? How can your packaging address those needs and stand out from the competition?
Once you clearly understand your audience, you can incorporate the design elements we’ve discussed. Consider using bold, bright colours, clear and easy-to-read typography, compelling imagery, unique packaging shapes, and consistent branding.
It’s also important to communicate key information clearly and concisely. What is your product? What are the key benefits or features? Why should consumers choose your product over the competition?
Finally, don’t be afraid to be creative and have fun with your packaging design. Consumers are drawn to brands that have personalities and stand out from the crowd. By incorporating unique design elements and messaging that reflect your brand’s personality and values, you can create packaging that resonates with your target audience and leads to increased sales.
Testing Your Packaging Design
After you’ve invested time and resources into creating effective packaging, testing your design to ensure it resonates with your target audience is important. Here are a few methods for testing your packaging design:
- Surveys: One of the simplest ways to test your packaging design is to survey your target audience. You can show them different packaging designs and ask for feedback on their preferred design and why. This can provide valuable insights into what design elements are most appealing to your audience.
- Focus groups: Conducting a focus group is another effective method for testing your packaging design. This involves bringing together a group of individuals from your target audience and showing them your packaging design. You can then ask for their feedback on what they like and don’t like about the design and what changes they would suggest.
- A/B testing: A/B testing involves creating two different versions of your packaging design and testing them against each other to see which performs better. This can be done through online surveys or by conducting in-store tests.
- Eye-tracking studies: Eye-tracking studies can provide valuable insights into how shoppers interact with your packaging design. Eye-tracking technology lets you see which design elements attract the most attention and how shoppers scan the package for information.
Testing your packaging design ensures that it resonates with your target audience and leads to increased sales. This investment in testing can ultimately save you time and money in the long run by ensuring that your packaging design is effective before it goes to market.
Adapting Your Packaging Design Over Time
Even the most effective packaging designs may need to be adapted over time to stay relevant and resonant with your target audience. Here are a few reasons why you may need to adapt your packaging design:
- Changes in consumer preferences: Consumer preferences and values can change over time, which may require you to adapt your packaging design to stay relevant. For example, if consumers become more concerned about sustainability, you may need to incorporate eco-friendly packaging materials into your design.
- Changes in the competitive landscape: Your competitors may change their packaging designs, requiring you to adapt your design to stand out from the crowd. Keeping an eye on your competitors and their packaging designs can help you stay ahead of the curve.
- New product features or benefits: If your product evolves and offers new features or benefits, you may need to update your packaging design to communicate those changes effectively.
- New marketing strategies: If you change your marketing strategy, you may need to adapt your packaging design to align with those changes. For example, if you shift your focus to a new target audience, you may need to adapt your packaging design to appeal to that audience.
Packaging design captures consumers’ attention, communicates key information, and drives sales. By understanding the psychology of shopping behaviour, utilising eye-tracking studies, and incorporating key design elements, you can create packaging that stands out on the shelf and resonates with your target audience.
Starting with a deep understanding of your target audience, you can incorporate design elements such as bold colours, easy-to-read typography, compelling imagery, unique packaging shapes, and consistent branding to create effective packaging. Communication of key information clearly and concisely is important, as is creativity and personality in your design to stand out from the competition.
Testing your packaging design using surveys, focus groups, A/B testing, and eye-tracking studies is essential to ensure it resonates with your target audience. Regular evaluation and adaptation of your packaging design can help you stay relevant and effective over time.
By investing in effective packaging design, you can set your product apart from the competition and increase your chances of success in the competitive world of retail. So, take the time to invest in your packaging research and design, and watch as your sales soar.
Kadence International has expertise with the world’s leading brands in package testing. Get in touch or submit a research brief.